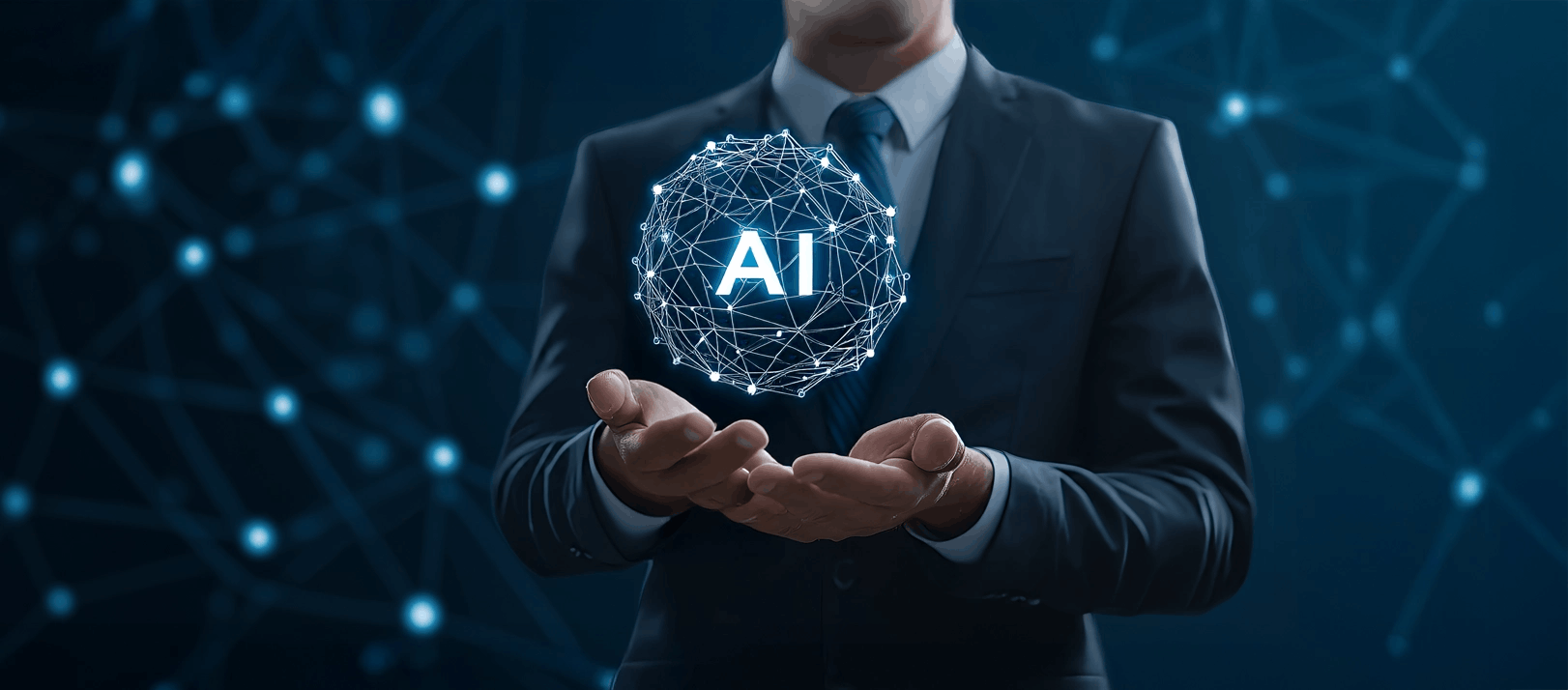
Being a savvy traveller, you are chatting with the new AI-powered customer support chatbot on the airline's app about your missed flight and need to board an alternate flight to your destination now. But does the chatbot know enough about you and your situation to help with your next move?
Generative AI for enterprise automation with RAG as its sidekick has a high potential for valuable use cases like this. But are you using Large Language Models (LLMs) in enterprises with traditional RAGs for customer-facing applications in production, or are you still stuck in the experimental phase?
RAG-integrated LLMs are smart on general knowledge but not on your business and what's happening in real time. Customers make real-time decisions, which means the window of opportunity for revenue and retention is real-time.
Context is the information a model uses to understand a situation and make decisions. This means the context the LLM receives needs to be appropriate, accurate, and real-time. Context can take various forms, including features, numerical data encodings, engineered prompts, etc. Further in this blog, we will discover why businesses must opt for AI-powered Agentic RAG enterprise solutions.
Retrieval Augmented Generation (RAG) is an innovative architecture that enhances the capabilities of large language models by combining information retrieval with language generation. In a RAG system, a retriever component fetches relevant data from a knowledge base, which is then utilized by a generator—typically a large language model (LLM)—to produce informed and contextually relevant responses. This dual approach allows RAG systems to deliver more accurate and reliable answers to complex queries, making it invaluable as an enterprise agentic RAG solution for search, data analysis, and customer support applications. By leveraging both retrieval and generation, RAG systems can handle various information retrieval tasks, providing enterprises with the relevant data they need to make informed decisions.
A passive assistant retrieves information in response to specific queries but cannot make proactive decisions. This means that the user is required to provide relevant data to generate responses.
A system that can handle straightforward queries but struggles with a complex query that seeks deeper contextual understanding or the decomposition of tasks. Its architecture is generally more straightforward and cannot adapt dynamically to changing information or user needs.
The next-gen Retrieval Augmented Generation is Agentic RAG, fabled for its proactive capabilities. It incorporates advanced data processing techniques to handle complex information retrieval tasks. It combines intelligent agents that independently make real-time decisions and enhance the system's ability to handle complex information retrieval tasks, thus allowing itself to act autonomously in decision-making processes.
Agentic RAG systems can adapt to various contexts and analyze data to provide strategic insights, making them suitable for a more sophisticated interaction with users and a better understanding of the relevance of information.
The key difference between Traditional RAG and Agentic RAG lies in their operational dynamics. While traditional RAG is reactive and limited to straightforward queries, Agentic RAG is proactive, capable of making autonomous decisions and adapting to complex scenarios, Thus making Agentic RAG a more versatile option in AI-driven information retrieval. Now that we know how these two RAGs differ in capabilities and usability let's look at all the possible reasons why this happened in the first place.
Traditional Retrieval-Augmented Generation (RAG) is no longer sufficient for enterprises due to its limitations in addressing the growing complexity of enterprise needs, data dynamics, and operational demands. Traditional RAG systems often rely on a single embedding model, which limits their ability to provide contextually relevant results. Here are the key reasons:
Traditional RAG relies on keyword-based searches or static pre-trained models, often failing to provide contextually relevant and comprehensive results. Unlike these conventional methods, semantic search transforms raw text into searchable vectors, enabling a nuanced understanding of queries and enhancing the relevance and accuracy of retrieved information. Enterprises require systems that dynamically adapt to new information and deliver precise, context-aware answers.
Static models also risk “hallucinations” (fabricated outputs) when they rely solely on outdated or incomplete training data. This makes them less reliable for critical decision-making.
Enterprises operate in environments where data is constantly updated. Traditional RAG systems struggle to integrate real-time updates without retraining the model, which is resource-intensive and time-consuming.
AI-powered agentic RAG business solutions allow integration with external databases that can be updated independently, ensuring responses are always based on fresh, accurate data.
Implementing RAG systems can be complex, requiring seamless integration with enterprise data infrastructures. Traditional RAG systems often struggle to integrate multiple embedding models, which limits their flexibility and scalability. Traditional RAG solutions may not offer the flexibility or scalability needed to align with diverse enterprise ecosystems.
Traditional RAG systems often require significant manual effort for data retrieval across multiple systems, leading to inefficiencies. Traditional RAG systems often lack advanced knowledge retrieval capabilities, which limits their ability to handle complex enterprise use cases. Enterprises need solutions that automate these processes, reducing manual labour and optimizing resource allocation.
For example, integrating RAG with tools like CRMs or knowledge management platforms can streamline workflows and enhance productivity by automating complex search tasks.
Customizing traditional RAG models for domain-specific applications often involves expensive retraining and fine-tuning. This approach becomes unsustainable as enterprises scale or update their data frequently. Modern RAG solutions leverage vector databases to reduce costs and maintenance challenges, making them more efficient and scalable.
AI-powered agentic RAG enterprise solutions are more cost-effective, as they leverage external knowledge bases without requiring extensive customization or retraining.
In fast-paced industries, enterprises need real-time insights to make agile decisions. Advanced RAG implementations utilise vector search to analyze diverse data sources and drive better decision-making quickly. Traditional RAG systems cannot provide real-time actionable intelligence from large, unstructured datasets.
Advanced RAG implementations enable enterprises to quickly analyze diverse data sources—such as customer interactions or market trends—driving better decision-making.
As consumers demand faster and more accurate services, enterprises face pressure to deliver instant results. Traditional RAG systems often rely on keyword search, which limits their ability to meet evolving enterprise expectations due to their limited adaptability and slower response times than modern AI-driven solutions.
As advancements in language models continue, the constraints imposed by token limits are diminishing. Traditional RAG systems often struggle to retrieve relevant documents due to token limitations, relying heavily on selectively retrieving information from external databases. This may not be necessary as models evolve to handle larger contexts and more complex reasoning without needing external data.
Many RAG systems utilize a fixed number of top-scored chunks to feed into the generative model. Traditional RAG systems often rely on a single embedding model, which limits their ability to provide nuanced and comprehensive insights. This approach can lead to inefficiencies, especially if the retrieved chunks are not dense with relevant information. As enterprises require more nuanced and comprehensive insights, the rigid structure of traditional RAG may fall short.
As enterprises explore more sophisticated applications of AI, such as intelligent search and advanced question answering, traditional RAG systems often struggle to integrate multiple embedding models, which limits their ability to provide a holistic approach to data utilization and AI capabilities.
There is a growing demand for integrated platforms that can provide a more holistic approach to data utilization and AI capabilities.
Curious about building your AI agent? We can help.
Modern RAG architectures have been developed to overcome traditional RAG systems' limitations. These include Retrieve-and-Rerank, Multimodal RAG, and Graph RAG. The Retrieve-and-Rerank architecture employs a two-stage process: an initial retrieval phase followed by a sophisticated reranking phase, which enhances response accuracy by selecting the most relevant information. Multimodal RAG extends the capabilities of traditional RAG by handling various types of content, such as images, charts, code snippets, and structured data, thereby offering a more comprehensive understanding of complex queries. Graph RAG, on the other hand, represents knowledge as an interconnected graph, enabling the system to grasp relationships and hierarchies within the information. These advanced architectures significantly improve the performance and versatility of RAG systems, making them more suitable for complex enterprise use cases.
In summary, while traditional RAG drawbacks have been a concern, they have proved their value for enterprise search and retrieval. Traditional RAG systems often rely on a single embedding model, which limits their ability to provide contextually relevant results. These systems have played a significant role in enhancing enterprise AI applications, but their limitations are becoming increasingly apparent. As enterprises seek more dynamic, flexible, and comprehensive solutions, the need for advanced Agentic RAG systems that adapt to evolving requirements becomes critical.
Embracing these advancements will be essential for enterprises to maintain a competitive edge in today's data-driven landscape. Advanced RAG solutions address these gaps by offering contextual understanding, automation, cost efficiency, and adaptability to evolving business environments.
We at Codiste understand the need to help businesses upgrade themselves with the newest advancements. Hence, we developed our enterprise agentic RAG solutions, which can be the game changer. To know more, connect with us today.
Share your project details with us, including its scope, deadlines, and any business hurdles you need help with.
Countries Served Globally
Technocrat Clients
Repeat Client Rate