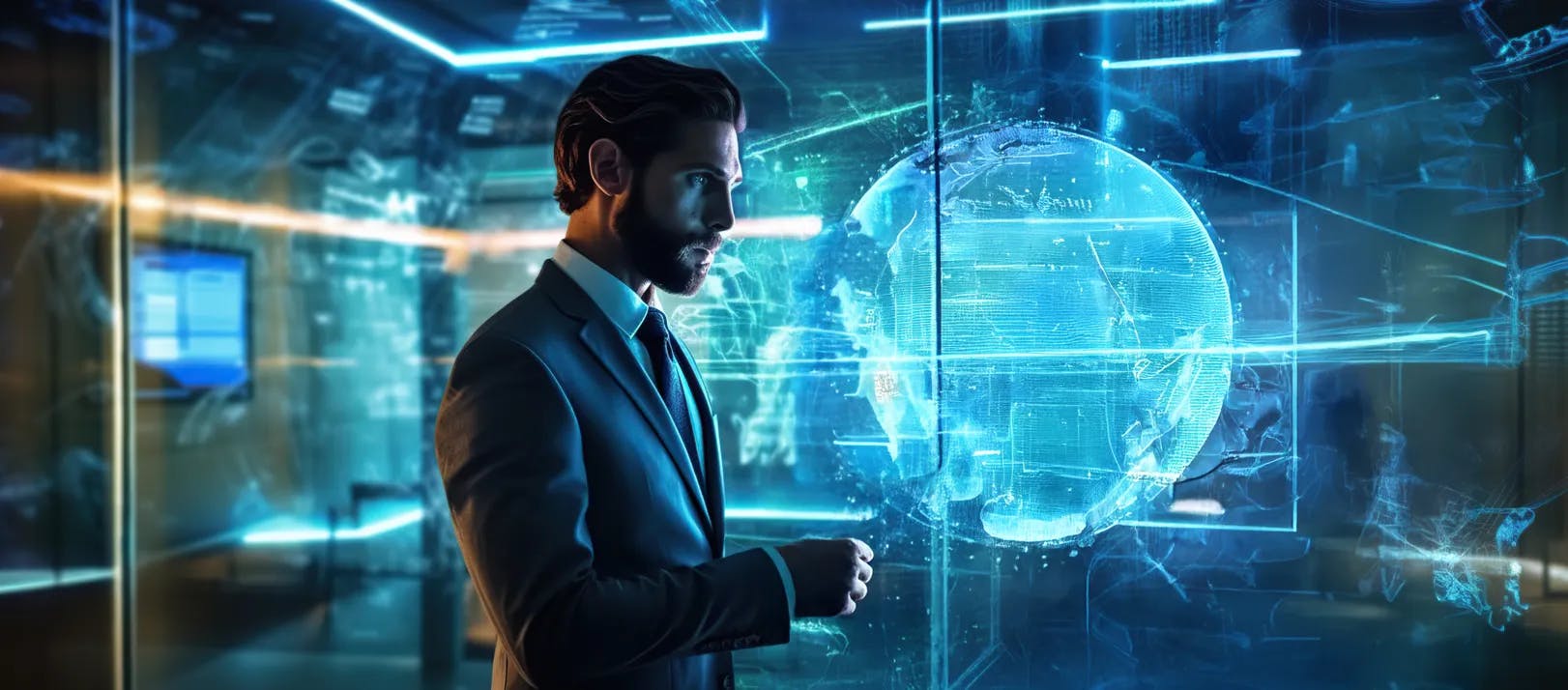
Artificial intelligence has made sure that modern businesses are evolving and open to a more utilitarian approach as these entities are increasingly turning to advanced solutions that can elevate business processes and automate workflows. Out of these lots, one is the retrieval agent known as Agentic Retrieval-Augmented Generation (RAG).
To stay competitive, businesses combine the precision of retrieval systems with autonomous decision-making; agentic RAG transforms how organizations process data, interact with customers, and innovate.
AI agent development has shown how agents can be utilized but also has the cost factor looming over, and the same can be said about agentic RAG development. Due to its significant upfront costs, many leaders wonder: Is this technology worth the investment?
Let’s break down Agentic RAG pricing models, the costs involved, potential ROI, and strategic value to help you decide. But before we proceed, let’s take a look at a few important and basic concepts.
Agentic RAG is a progressive AI framework that perfectly integrates retrieval mechanisms with generative models to produce precise and relevant outputs, unlike traditional generative AI models. Agentic RAG enables AI agents to access and pull from external knowledge sources before generating content. This ensures that the responses are not only accurate but also informed by the most up-to-date and relevant information available.
Before we move further into the nitty gritty of Agentic RAG pricing models, let's take a look at what constitutes RAG.
Retrieval-augmented generation (RAG) leverages external knowledge sources to help generative AI function. At its core, RAG fetches and incorporates factual or contextual information to enhance the accuracy of responses, effectively addressing the limitations of traditional LLMs that may lack real-time updates or external knowledge.
The evolution of RAG can be categorized into three distinct phases:
RAG systems provide more accurate and contextually relevant responses by leveraging external knowledge sources, making them invaluable for applications that require up-to-date information and nuanced understanding.
AI agents are computational entities designed to perceive and respond to their environment using intelligent processes. When a multi-agent architecture like Agentic RAG is involved, these agents play a crucial role in tasks that involve sequential decision-making, flexibility, and planning. They utilize Large Language Models (LLMs) as decision-makers to determine the next best action, allowing them to take multiple non-linear steps to achieve a specific goal.
One of the standouts features of AI agents in Agentic RAG is their ability to function independently. They can generate responses based solely on input queries without relying on external dependencies, making them highly versatile and efficient.
Further below, we take a look at the agentic RAG architecture as an overview to understand its functionality.
The architecture of Agentic RAG systems is designed to combine the strengths of RAG and AI agents, creating a robust and versatile framework for LLM-powered applications. This architecture involves incorporating one or more types of AI agents into RAG systems, enabling the creation of more powerful and adaptable solutions.
Agentic RAG systems can include various types of AI agents, such as:
These agents work together to retrieve information from various sources, including vector databases and other tools.
Unlike traditional RAG systems, which rely on static workflows, Agentic RAG dynamically adapts to user needs, delivering highly accurate, context-aware responses. Agentic RAG’s AI framework merges retrieval mechanisms (fetching relevant data from vast datasets) with autonomous agents capable of reasoning, learning, and acting on that data. This technology is ideal for applications requiring:
An agentic RAG system further enhances these capabilities by incorporating a retrieval component along with AI agents, enabling more adaptive, accurate, and scalable data retrieval and reasoning.
Business Process Automation Made Easy with Agentic RAG, Try Yours Now!
Before diving into ROI, it’s critical to understand the investment required. Here’s a detailed cost analysis:
Implementing Agentic RAG involves setting up a rag pipeline, which incorporates various agents to enhance information retrieval and response generation.
The initial setup costs can be high, especially when considering the integration of relevant data sources. These sources are crucial for ensuring that the RAG pipeline utilizes optimal information to enhance query handling and overall system performance.
The true power of Agentic RAG lies in its ability to drive measurable returns by integrating relevant context. Here’s how:
The answer is entirely subjective and truly depends on your organization’s needs:
If your company frequently deals with complex queries and requires highly accurate and versatile responses, investing in Agentic RAG could be beneficial. The integration of intelligent agents within RAG pipelines enhances the system's ability to manage queries, select appropriate tools, and adaptively retrieve data from multiple sources, leading to improved response quality.
Agentic RAG isn’t just another tech fad! It’s a global strategic phenomenon coming as an advantage for businesses ready to harness AI’s full potential. By integrating relevant context from external knowledge sources, Enterprises opting for agentic RAG without giving heed to agentic RAG pricing models will certainly enhance the performance of LLM-powered applications by integrating external tools, reducing inaccuracies and improving user query results. While initial costs for agentic RAG development are steep, the long-term benefits in efficiency, customer loyalty, and innovation make it a compelling investment.
If you're planning to build smart AI agents, Codiste can help. We specialize in agentic RAG development and have hands-on experience in creating intelligent, tool-integrated AI systems that deliver real value.
Share your project details with us, including its scope, deadlines, and any business hurdles you need help with.
Countries Served Globally
Technocrat Clients
Repeat Client Rate