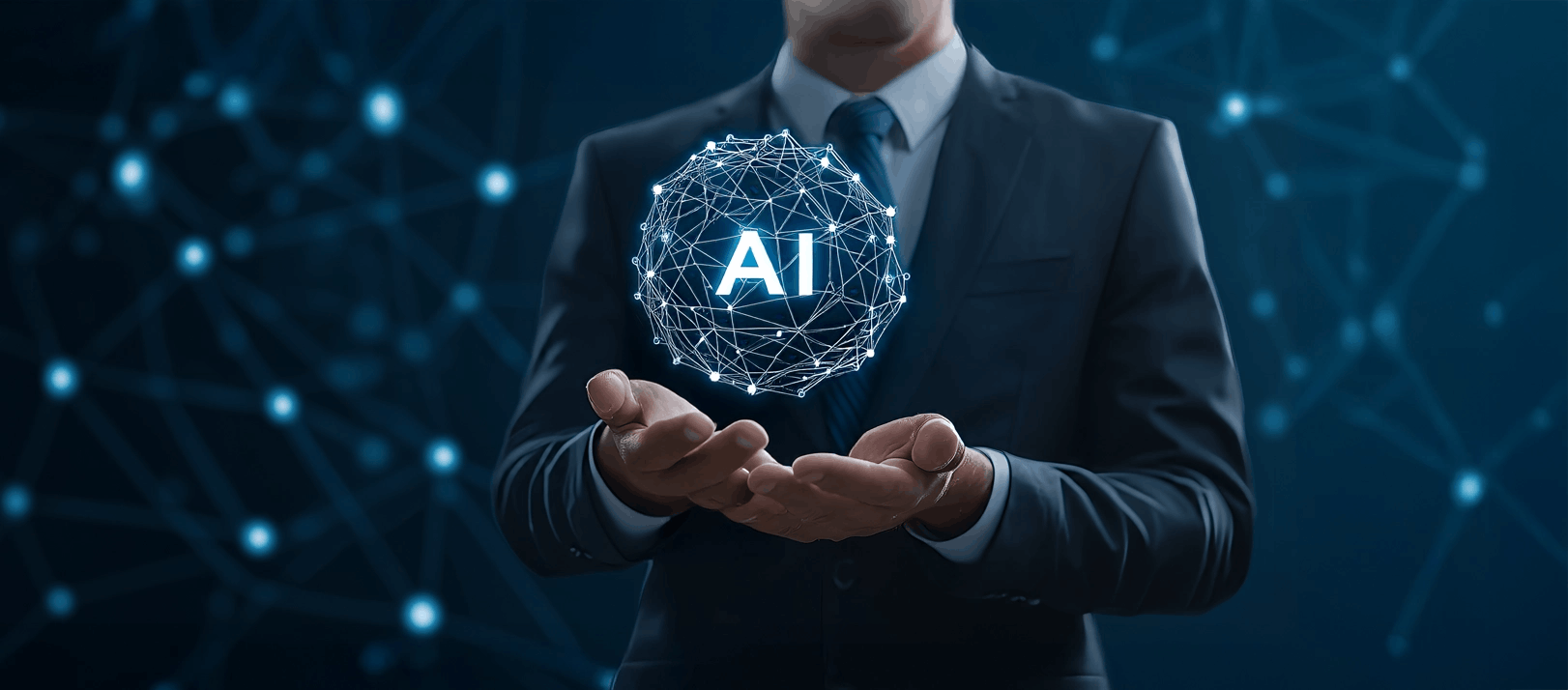
In the modern age, enterprises are flooded with huge amounts of data daily. This large amount of data makes it difficult for companies to find relevant information. Traditional Retrieval-Augmented Generation (RAG) models solve the problem by retrieving useful data and then generating responses. However, they have certain drawbacks. They often need human intervention and can’t handle tasks requiring more than one step.
The solution to the issue is found in a new player, Agentic RAG. This leading-edge method brings together AI-based automation and smart decision-making that aim to boost data retrieval and analysis. Enterprises using Agentic RAG automation are capable of making high-value decisions much quicker and more precisely without having to constantly use human labor.
This article explains the functioning of the Agentic RAG, its advantages, uses and difficulties. It also brings you up to speed with the technology, which is growing exponentially as organizations manage growing data.
The RAG (Retrieval-Augmented Generation) technique correlates with the improvement of AI models by linking them with external data sources. The following two steps are followed by this process:
Traditional RAG cannot, however, act alone since it possesses no decision-making abilities and, therefore, is limited in complexity but can complete pre-defined tasks.
Agentic is a term that is used to describe AI systems that behave like intelligent agents. These agents can:
Agentic RAG, which can think, plan, and improve independently, is essentially a smarter form of RAG. As opposed to traditional RAG models that solely acquire and produce text-based responses, Agentic RAG automation takes a larger stride in terms of independently analyzing the data, adopting its methods and boosting efficiency without human intervention.
Agentic RAG comprises several highly advanced AI capabilities:
All these essentials collaborate to develop an independent data analysis system. AI-driven Agentic RAG business solutions are perfect for companies looking to make their operations efficient.
Agentic RAG is a method that brings several advantages over traditional RAG and manual data analysis:
One of the critical things that a financial professional needs to rely on when it comes to investment decisions is market data. Agentic RAG for data retrieval can look through several reports, news, and the company's financial statements quickly, all while providing you with thorough summaries and insights. As a result, such applications support investors, financial analysts, and banks in making intelligent decisions. They do not have to spend significant time manually going through the market trends.
The financial sector is constantly at risk. The usage of Automated data retrieval analysis can be one of the ways to help through the analysis of real-time data. This will help to detect fluctuations in the market and notify companies in advance. Not only that, it can also predict risks based on past information and give the companies the time to get ready and to be ahead of potential financial issues.
It is common for researchers to review large volumes of literature. AI-powered Agentic RAG enterprise solutions speed up the process of summarizing academic papers and detecting the main points with the use of automation of data retrieval. AI facilitates scientists to summarize these papers quickly and helps them to focus more on the most important findings. In other words, instead of researchers going through hundreds of studies with their own hands to find the relevant data, they can use AI-powered Agentic RAG enterprise solutions to do it with multiple sources.
AI-powered Agentic RAG enterprise solutions can take existing research data that have been collected, analyze the data, identify patterns, and even suggest new hypotheses for further exploration. This substantially accelerates the research which in turn makes sure the scientists have the most significant information available to them.
Customer support teams have to handle multiple queries on a daily basis. One of the ways chatbots get the right information from company knowledge bases is by processing enterprise data. Chatbots can analyze common issues or give solutions in real time. It helps to reduce the number of human interventions, thus enabling improved customer satisfaction.
Using prior interactions as a reference, AI can learn. This means that companies can use AI that can predict the most frequent customer complaints and propose relevant solutions to them in advance. This, in turn, will make customer service better and will be a great asset in helping to reduce the duration of solving the issue.
Companies provide sales, marketing, and operations departments with various reports. Through AI-driven data analysis, this data can be compiled with less human intervention, which certainly optimizes decision-making. Companies can get ready-made reports that are well-arranged and require no input from a human to carry out every step.
Instead of combing through several documents, employees are now able to solely use Agentic RAG for data retrieval to immediately fetch the necessary files from company archives. As a result, employees get the information they need quicker and workplace productivity goes up.
Agentic RAG automation can be of great help to software engineers and data analysts in the following ways:
It enables them to debug codes by retrieving the relevant documentation and tips.
It allows them to explore large datasets for trends and insights.
Repetitive data-processing tasks can be automated, which in turn saves the developers time to focus more on their innovative ideas.
Even though Agentic RAG is a useful tool, there are a couple of problems to think over:
Looking ahead, the future of AI-powered Agentic RAG enterprise solutions will likely involve:
Currently, we see more data-driven technologies in the world. Agentic RAG Automation is one of those innovations that is changing the way companies get and process information. By deploying smart AI agents, enterprises can do less manual work, systematize decision-making processes, and get a leg up over competitors.
If you are looking for an advanced AI-powered data analysis solution for your business, then Codiste can help. AI-driven data automation is the way forward for every growing enterprise. Codiste, through their well-versed implementation of Agentic RAG can produce solutions suited to your unique needs.
Contact us today for assistance with automatically retrieving and analyzing data and you will see the impact it can make in your company!
Share your project details with us, including its scope, deadlines, and any business hurdles you need help with.
Countries Served Globally
Technocrat Clients
Repeat Client Rate