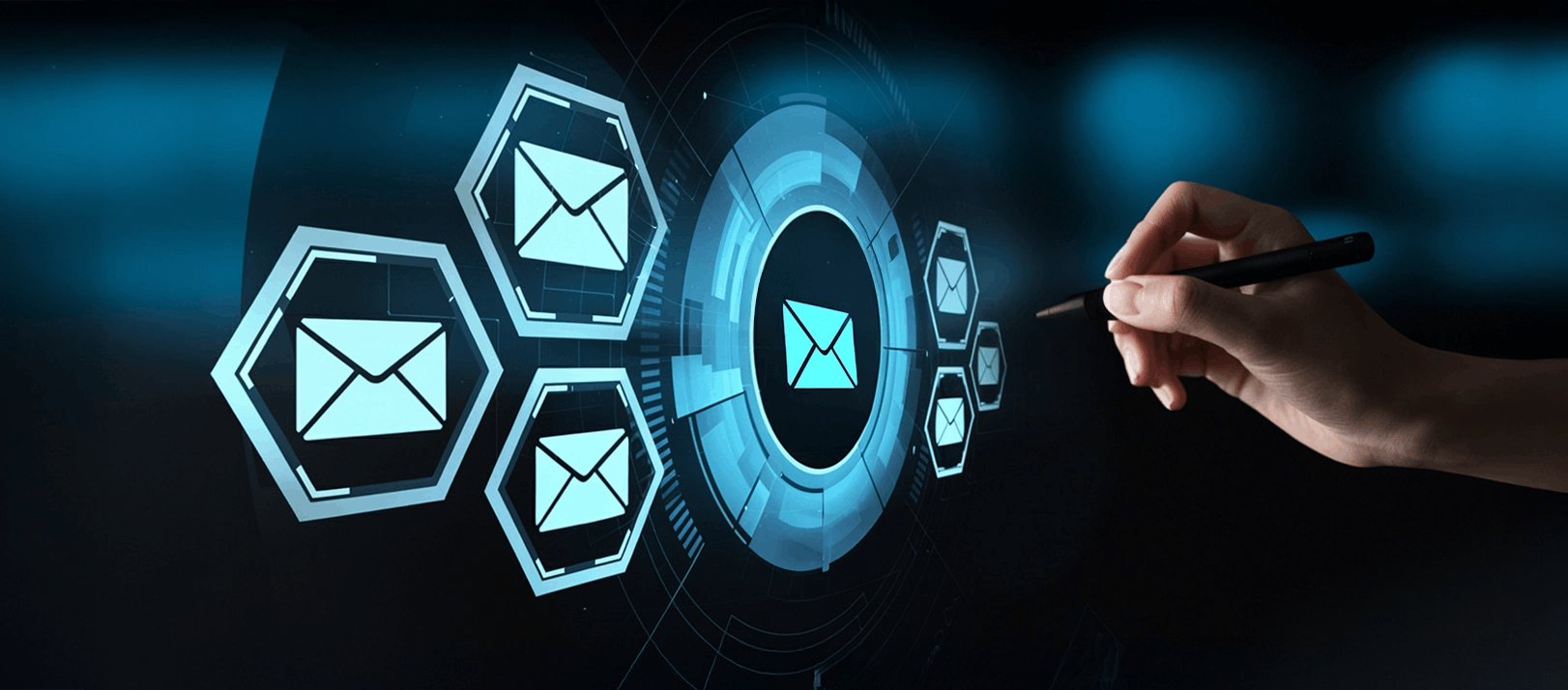
The AI community is abuzz with discussions about the new DeepSeek R1 AI model, which is reshaping how we think about efficient AI training. Developed by DeepSeek, a Chinese AI startup originating from the High-Flyer hedge fund, this model demonstrates exceptional reasoning capabilities.
DeepSeek R1 performance is comparable to OpenAI's o1 series on key AI model benchmarks, and its distilled 7B version even outperforms larger open-source models. However, beyond just raw power, DeepSeek hints at a more profound transformation: a future where domain expertise plays a more significant role than sheer computational scale in AI expertise development.
What makes the DeepSeek R1 AI model particularly interesting is how it achieved strong AI reasoning capabilities. Instead of relying on expensive human-labeled datasets or massive computing, the team focused on two key innovations: Automated Verification for Training Data – Focusing on domains like mathematics where correctness is unambiguous.
Optimized Reward Functions – Efficiently identifying which new training examples would improve the model, avoiding wasted computing on redundant data and cost-effective training.
The DeepSeek models, particularly DeepSeek-R1-32B and DeepSeek-V3, demonstrate impressive performance across a range of benchmarks, highlighting their advanced capabilities in various AI tasks. In a recent GitHub survey, in terms of accuracy, DeepSeek-R1-32B achieves notable scores of 79.8% and 79.2%, outperforming other models and showcasing its reliability in precision-driven tasks.
When it comes to competitive programming, as measured by the Codeforces benchmark, DeepSeek-R1-32B excels with a 93.4% percentile, the highest among the listed models, indicating its strong problem-solving skills in complex coding challenges.
Additionally, in the GPQA Diamond benchmark, which tests complex question-answering abilities, DeepSeek-R1-32B achieves outstanding scores of 97.3% and 96.4%, further underscoring its proficiency in handling intricate and specialized queries. While its performance in the MMLU (Multitask Language Understanding) benchmark is relatively lower at 49.2% and 48.9%, it remains competitive in multitask learning scenarios.
Although specific data for the SWE-bench Verified (Resolved) benchmark is not provided, the model's strong performance in other areas suggests robust capabilities in software engineering tasks. Overall, DeepSeek-R1-32B and DeepSeek-V3 stand out as highly effective AI models, particularly in accuracy, programming, and advanced question-answering, making them strong contenders in the field of expertise-driven AI.
Application developers now have access to powerful, open-source models upon which to build. DeepSeek R1 capabilities offer a solid foundation for those wanting to develop intelligent applications, including AI application development, without needing to invest in costly model training. The potential for creating AI-powered apps using DeepSeek R1 models can significantly enhance business efficiency and reduce costs.
DeepSeek’s open-source release of six smaller models—ranging from 1.5B to 70B parameters—gives developers flexibility in choosing the right balance of size and efficiency for their needs. Their distilled 14B model, in particular, outperforms more significant open-source alternatives on key AI model benchmarks, making it an attractive option for AI application builders.
For major AI labs, DeepSeek's efficiency techniques will not slow the race for larger models but will accelerate it. These innovations will be leveraged alongside massive computational resources to advance general-purpose AI further.
The computing race at the top will continue but with better fuel. By using DeepSeek's approach to optimizing training resources, major AI labs can enhance training processes in efficiency and performance, allowing for faster iteration cycles and breakthroughs in AI model advancement.
The most intriguing implication of DeepSeek R1 performance is its potential to empower domain experts. Traditionally, startups and enterprises have been advised to build on top of existing AI models rather than developing their own. However, DeepSeek demonstrates that with the right expertise, it’s possible to create highly optimized, specialized AI models using only modest computing resources.
Developing custom AI models, especially for tasks like personalized recommendations and advanced natural language processing, typically takes between 3 to 12 months. This timeline is crucial due to the complexity involved in data collection, model design, and continuous post-launch investment in language models.
Industrial operations, where real-world performance data creates feedback loops.Data acquisition and access to relevant data are crucial for developing accurate AI models, as they significantly influence both the model and learning process and overall costs.
By leveraging DeepSeek’s training methodology, domain experts can:
The power of potent machine learning systems is now just a click away efficient, scalable and robust.
The comparison of DeepSeek R1 vs GPT-4 raises interesting questions about the future of AI expertise. While GPT-4 remains a powerhouse in general intelligence, DeepSeek’s approach suggests that in specialized domains, more innovative training could be just as crucial as larger model sizes. The model’s success hints at a shift in focus from raw computing power to domain-driven innovation.
The development process of AI software, such as DeepSeek R1 and GPT-4, varies significantly based on the resources and expertise of the companies involved. This process emphasizes the importance of ethics, bias, and regulatory compliance, along with the need for transparency to navigate the evolving legal landscape.
DeepSeek R1’s efficiency-first training methodology challenges the conventional belief that AI progress is primarily tied to increasing parameter counts. Instead, it underscores the role of targeted learning and domain-specific AI enhancements in achieving high-level AI capabilities with fewer resources.
Application developers leveraging increasingly powerful open-source models.AI labs using DeepSeek-style efficiency techniques to train even larger general-purpose models.
Domain experts apply these methods to build specialized AI models with a fraction of the resources previously required.
This third category—where domain expertise meets optimized AI training—could be the most transformative. DeepSeek R1 review suggests that innovative training techniques, rather than just computational power, could define the next wave of AI breakthroughs. The industry is on the verge of a significant shift, and DeepSeek R1 has paved the way for others to follow, each applying these methods to their unique fields.
AI technologies also have the potential to optimize supply chain and inventory management, enhancing operational efficiency by automating logistics and reducing costs, particularly in manufacturing and transportation.
As AI technology evolution continues, the key question remains: Will more competent training surpass raw computing power? If DeepSeek’s success is any indication, the answer might be closer than we think. With rapid AI industry developments, the emphasis on AI reasoning capabilities and AI model advancement is becoming increasingly important. The future of AI expertise lies in those who can integrate domain knowledge with AI efficiency, paving the way for a more specialized, performance-driven AI landscape.
Looking to transform your business with efficient AI & ML solutions? Let us help you unlock smarter, more enhanced AI models tailored to your needs—boosting performance without the need for endless computational power. Start your journey today
Share your project details with us, including its scope, deadlines, and any business hurdles you need help with.
Countries Served Globally
Technocrat Clients
Repeat Client Rate