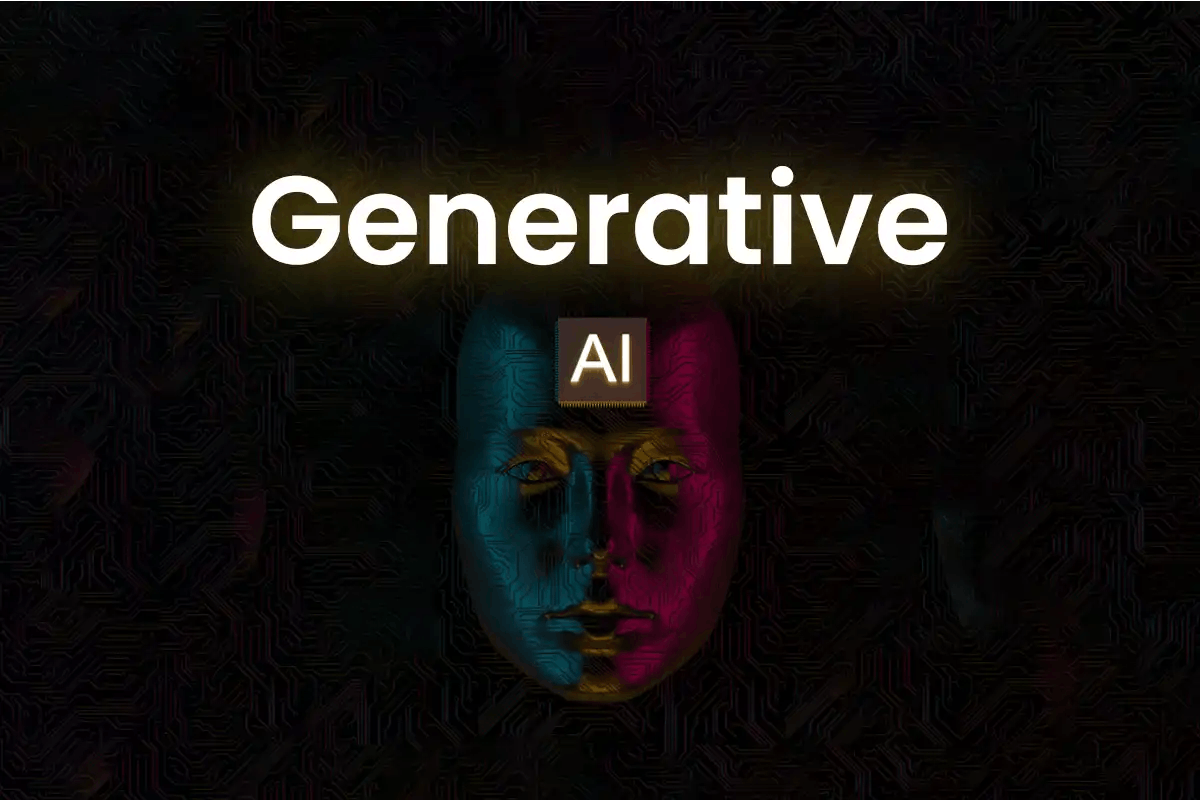
Recent years have seen artificial intelligence or AI make huge strides. They have now reached a point where they can create quite sophisticated and innovative pieces of art. Be it pictures or tunes, poetry, and beyond, AI models are steadily reducing the gap. We once thought this gap between tech and imagination could never be bridged.
These AI art systems use neural networks and deep learning. They train on huge collections of human artworks. This allows them to see intricate patterns people can't. Then they can create their new pieces with original style and creativity. The art can be odd or amazing. AI-generated arts are not flawless, but they show promise and going forward can become one of the biggest innovations. Generative AI art also opens up new questions about rights, ethics, and influence.
The starting point for any AI art system is gathering and preparing data on which the machine learning model will train. Artists and engineers work closely to finesse the network architectures and hyperparameters that influence creative output. As the models advance, collaborators guide the training with quantitative measures as well as qualitative human judgment.
Putting together a wide range of high-quality art data is key to training creative AI. The models need to see many examples of different styles and art forms, labelled accurately. Clean data without errors allows the models to learn patterns smoothly. The neural network brains need enough capacity to capture complex creative ideas. As training progresses, engineers and artists give feedback on the AI's outputs to focus on improvements and make sure it stays aligned with human expectations of creativity. AI systems progressively enhance their creative abilities with each round of training with diverse, accurate art data, robust models, and continuous human guidance.
Putting together lots of good art data is key to teaching AI to be creative. The models need to see many examples of different art styles, accurately labeled. Clean, error-free data allows the models to learn smoothly. As training progresses, engineers and artists give feedback to improve the AI and keep it creative.
The data to train AI art image models requires careful preparation first. Datasets have thousands to millions of images, audio clips, texts, or other multimedia elements. Humans meticulously clean, structure, and normalize the data so the patterns are clear for the AI to pick up. Curating diverse examples and styles allows more creative flexibility. Filtering ensures quality over quantity, as bad data can limit the AI’s credibility. The models effectively learn creativity from diverse examples with mindful data preprocessing.
Researchers pick smart neural network models to start with based on the art form. Options like GANs, VAE, or Transformers have shown creative promise before. These base architectures then train on the prepared data to optimize for originality and quality. Fine-tuning them this way results in AI that mixes content and style well.
Fine-tuning is key to making the AI models creatively strong. After the initial training, researchers further tweak the neural networks through more learning. This end-to-end adjustment teaches them the unique styles and qualities of each art type. Optimising the models this way allows them to generate novel pieces that feel more human-made. The fine-tuning process is what makes the AI art truly shine.
More training after the initial learning is key for creativity. Researchers further tweak the neural networks step-by-step this way. This teaches them the unique styles and qualities of each art form. Fine-tuning results in AI that makes novel artworks with a more human feel.
Humans work together with the AI when training it in art. Technical measures give some training feedback. But subjective human assessment of what makes creativity, emotion, and beauty is equally crucial. Artists collaborate with coders to judge new outputs and upgrade datasets for improvement. The symbiotic partnership between human and machine creativity helps both get better
Artificial intelligence can now autonomously create original, creative artworks. Powerful AI systems produce art comparable to or enhancing traditional art. However, as AI becomes more involved in human creativity, vital ethical questions around attribution emerge.
Thoughtful development is necessary to fully realise AI's capability for expanding human expression. If cultivated responsibly, AI art augments rather than compromises human creativity by expanding our collective appreciation of what both computer code and the human mind offer. There is tremendous creative capability and ethical risk in fusing organic and artificial intelligence. As the margin between creativity and code narrows, we must guide this technology to serve human expression.
Style transfer changes a machine learning model to adapt its output to new styles while keeping important content. AI art made in one style can be altered by adjusting model gradients and weights to mimic famous painters or musical genres. This grants the AI creative flexibility and range. For example, a landscape painted by the AI could be stylistically transformed to look like a Monet or Picasso with the core elements intact. Music could shift between classical compositions and jazz improvisations while maintaining the essential melody. Style transfer expands what an AI artist can creatively accomplish.
Algorithms can make AI art more interesting by increasing novelty and surprise. Methods like latent variable manipulation randomly tweak the internal workings of generative models to produce refreshing changes that still feel coherent. Other new techniques incorporate storytelling structure or let human collaborators directly refine outputs through easy editing tools. For example, an algorithm could take a basic AI-generated landscape painting and add an unexpected rainbow or hidden forest creature to make it more imaginative. Music could transform from a simple melody into a multidimensional orchestral piece. Algorithms boost AI art creativity.
AI art has many practical uses - in marketing, entertainment, gaming, and more. It can swiftly generate custom visuals to engage specific audiences. The technology assists musicians by accelerating creative tasks. Game developers employ AI tools to efficiently produce more content for players.
As AI art progresses, even more industries can benefit, like architecture, news media, and beyond. Firms may use it to quickly visualise designs. Publishers could instantly generate images for articles. The creative applications are far-reaching. Overall, this emerging technology brings practical capability to myriad real-world settings.
As AI art spreads, it brings up ethical issues around credit, bias, and effects on artists. Developers and policymakers need to address these issues proactively so that AI art grows thoughtfully.
There are valid worries that traditional artists may face money troubles if AI replacements come about quickly, capability jeopardizing careers. Policymakers should think about protections for human creatives moving forward. But if guided properly with care and responsibility, AI art can still thrive alongside human art rather than push it aside completely.
For example, AI tools may best amplify rather than replace creative roles - assisting human artists with ideation, composition, production tasks and more to increase efficiency. With ethical development principles and a collaborative spirit, AI stands to bolster artistry through interdependence rather than isolation. As this emerging technology continues maturing, striking the appropriate balance of oversight and freedom will help it blossom responsibly and for overall benefit.
AI models don't make art from scratch. They require a huge amount of human-made artworks to learn from. The creators of those works deserve proper credit. The models have so many inputs though that giving credit to everyone is hard. New rules are needed to correctly acknowledge the original artists, even if done anonymously or indirectly.
More openness and transparency about what goes into AI art would help address attribution challenges. Developers could better document how models are trained, what data is utilised, and how user inputs get translated. Detailed creation records would provide clearer acknowledgment pathways. As long as artwork origins stay opaque, confusion around ownership will persist. Striking the right balance regarding transparency will enable AI art to flourish fairly.
AI art systems also need to reduce biases that can show up in algorithms. Biases can come from issues in the original training data or from how the models are optimised. More research and responsible data sourcing are key to making sure AI art generation encourages inclusivity.
Companies creating AI art tools should place a high priority on auditing and mitigating algorithmic bias. They ought to regularly review training data and model outputs for problematic patterns that can marginalised or misrepresent minority groups or genders. Diverse technical teams, bias testing practices, and end-user rating systems can go a long way toward upholding equity and inclusiveness as AI art capabilities accelerate. Prioritising ethical development processes now will enable more trustworthy advancement of the technology.
Artificial intelligence can now learn what makes art creative and produce original, creative works. Powerful AI systems are creating artworks that compare to or enhance traditional art. However, as AI becomes more involved in human creativity, important ethical questions around attribution come up.
Moving forward thoughtfully is necessary to fully realise AI's capability for expanding human expression. If developed responsibly, AI art does not compromise human creativity but rather expands our collective appreciation of what both computer code and the human mind can contribute. There is as much creative capability as ethical risk in the fusion of organic and artificial intelligence. As the line between creativity and code blurs, we must guide this technology to serve human expression.
The democratisation of art gives more people a voice but can overwhelm traditionally underrepresented communities or discourage human artistic careers. Responsible AI art should not dominate distribution channels or profit from human creations without fair attribution or payment. Instead, technology can enhance human creativity into a mutually beneficial relationship.
The rapid evolution of AI art can be better understood through technical case studies spotlighting the architecture and performance of milestone systems that set new benchmarks for the state-of-the-art.
Artificial intelligence is increasingly able to learn what makes art creative and produce original, creative works. Powerful AI systems can now autonomously create artworks that rival or complement traditional art. However, as AI becomes more involved in human creativity, important ethical questions around attribution arise.
Looking ahead, teaming up is essential among computer scientists, artists, lawmakers, and the public. This is vital to explore the full capabilities of AI in boosting human expression. Companies like Codiste are leading the way in ethical and responsible AI development for creative applications. If developed thoughtfully, AI-generated art does not compromise human creativity but rather expands our collective appreciation of what both computer code and the human mind can contribute. The synergy between organic and artificial intelligence contains as much creative capability as ethical risk. As the line between creativity and code continues to blur, we must thoughtfully cultivate this technology in service of human expression.
Share your project details with us, including its scope, deadlines, and any business hurdles you need help with.
Countries Served Globally
Technocrat Clients
Repeat Client Rate