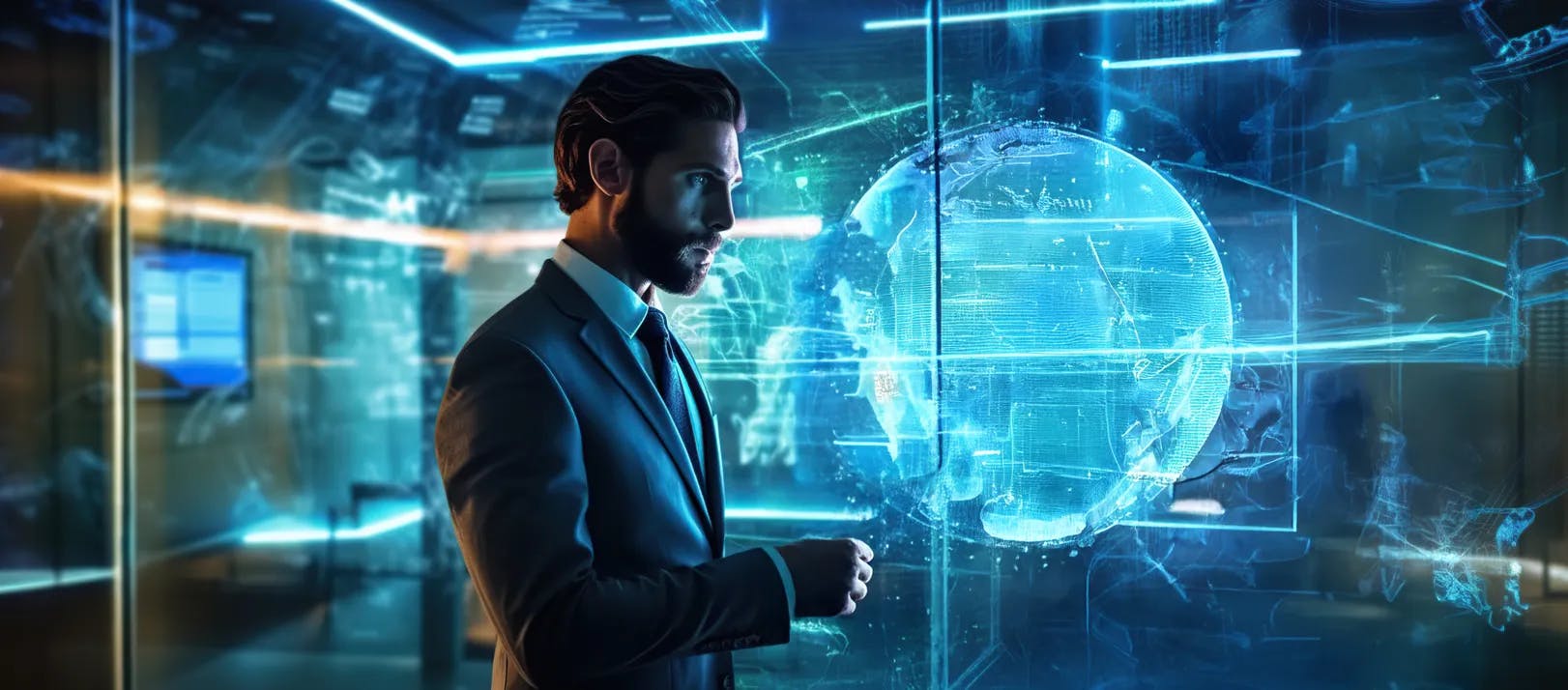
Scalable AI solutions for enterprises have been a total game changer in the way business does various things. It pushes the boundaries by helping automate, increasing effectiveness, and enhancing data-driven decision-making. Based on findings from the latest survey, the market for AI technology in enterprises is forecasted to rise to $68.9 billion by 2028, with a CAGR of 43.9%. This shows the major impact of AI across different sectors.
Nevertheless, the process of introducing AI into big enterprises has some major obstacles that include problems like data silos, scalability issues, real-time decision-making, and workflow complexity. Also, the ability of the system to work in new environments is one of the major issues.
Agentic Retrieval-Augmented Generation (Agentic RAG) was born as a unique option to tackle these Enterprise AI challenges. The technique improves the performance of business AI systems and provides correct, detailed, and real-time insights by combining AI-powered retrieval with autonomous agents.
In this article, we will deal with the five main pitfalls of the business AI field and how the innovative technology Agentic RAG for enterprise AI steps in to resolve these issues.
The Agentic RAG is a sophisticated AI model that is the advanced version of Retrieval-Augmented Generation (RAG). It is a means of incorporating artificial intelligence agents that can work on their own by proactive decision-making. Classic RAG systems improve the answers of AI by referring to the relevant data, which is in many cases a passive operation.
In contrast, Agentic RAG is associated with AI agents, who are capable of taking a more substantial role by retrieving, scanning, and directly responding to the information. This helps to create more responsive and intelligent AI systems.
Through intelligent agents, companies can easily implement AI automation that is more advanced than the basic level. The agentic qualities of RAG systems enable them to produce and implement a plan as well as modify the plan according to the actual situation based on real-time data. This perfectly suits them in the case of large high-tech companies that are trying to get rid of ambiguity and storage issues, which are oftentimes vague and enormous.
Also, the implementation of Agentic RAG solutions will offer more than a few benefits to companies:
Now, let's get into the details of the five major enterprise AI challenges and how Agentic RAG tackles them.
One of the Enterprise AI challenges with bigger companies is that they often have data stored in different systems that are not connected. It creates problems in accessing the data properly. This fragmentation is the critical thing that prevents AI from making decisions that are based on baseline information.
2. Scalability Issues
When organizations spread out quickly, the systems based on artificial intelligence often cannot function at scale effectively. Usually, the earlier models have to be extensively retrained, and the infrastructure also needs to be upgraded. This leads to an increase in costs and operational bottlenecks.
3. Real-Time Decision-Making Constraints
Every company needs AI models that can quickly respond to data and trends in a fast-moving market. The classical AI models are faced with the problem of latency when processing and analyzing big data sets.
4. Complex Workflow Management
Enterprises that depend on multi-step workflows, are composed of various departments and technologies. These workflows can be managed manually or with static AI models and this often causes mistakes and inefficiencies.
5. Adaptation to Changing Business Environments
Artificial intelligence (AI) models have to respond consistently to the changes in regulations, market trends and technology. Old-style AI systems demand the repetition of the training process, due to which the operational expenses increase.
The use of artificial intelligence technologies in companies is accompanied by numerous problems, from data fragmentation to scalability. However, the Agentic RAG's solutions seem to be a core of the solution where the AI systems are turned to be increasingly intelligent, scalable, and responsive. Moreover, companies can look at reducing operational expenses, using data for better decision-making, and staying in the lead in the market by the use of AI-powered Agentic RAG enterprise solutions.
Collaboration with experts is a must-have for companies that are considering AI automation for enterprises to improve their effectiveness. Codiste is an expert in building custom AI-based Agentic RAG enterprise solutions that provide agility, scalability, and adaptability. Our products are uniquely designed to handle issues that AI represents to an organization. They are built to fit seamlessly with the existing systems and over time be developed into human AI co-working systems.
Businesses can use Codiste to take advantage of the RAG for enterprise AI, thus moving from problems to turning them into possibilities. Reach out to us, so we can introduce to you the incredible power of Agentic RAG in the context of your business enterprise AI strategy.
Share your project details with us, including its scope, deadlines, and any business hurdles you need help with.
Countries Served Globally
Technocrat Clients
Repeat Client Rate