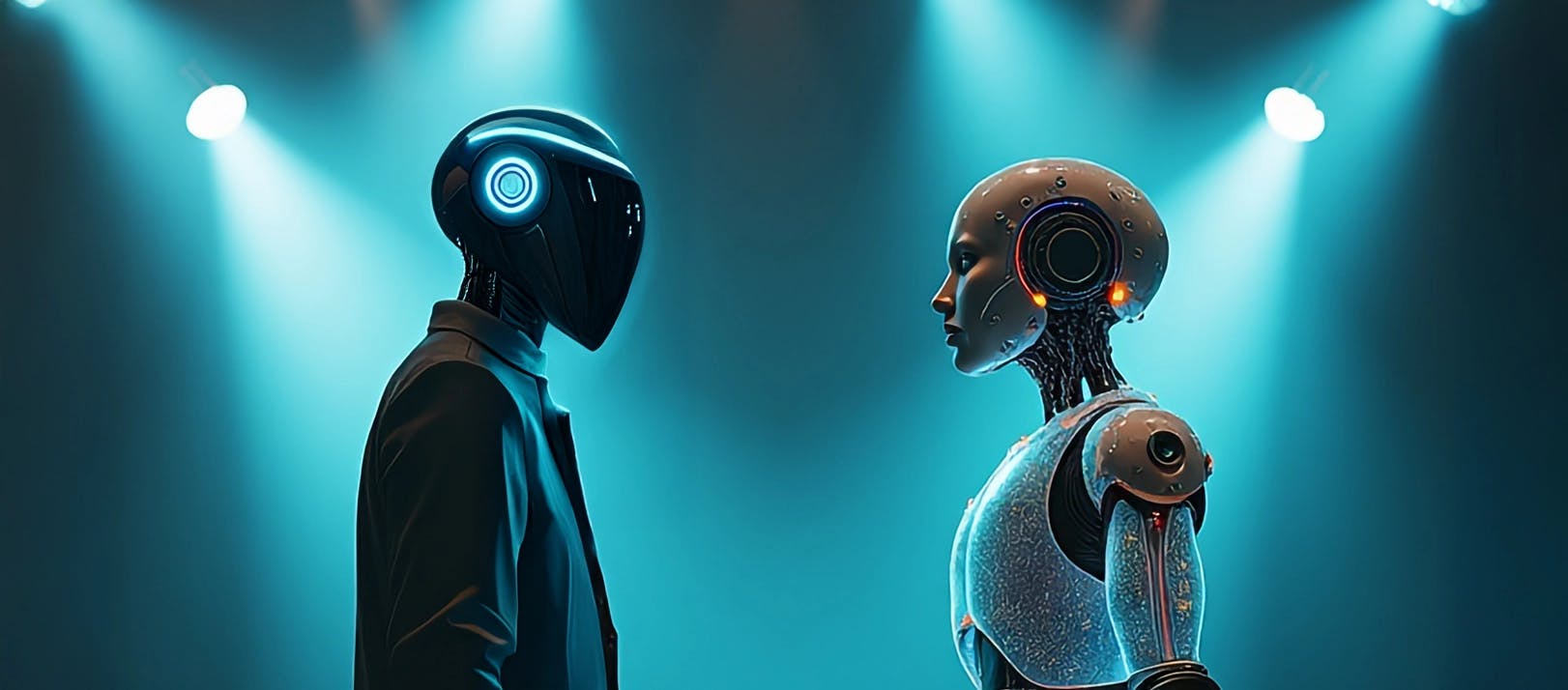
,
,
As enterprises increasingly seek AI to streamline operations, a new, reliant, adept frontier has emerged—Agentic AI. This next-generation AI goes beyond its traditional cousins, offering the power of deep integration, autonomous decision-making and data predictions in business operations. AI development has unlocked unparalleled opportunities for businesses to evolve and grow, from automating routine and complex tasks to driving quick, more thoughtful, data-informed decisions.
As a well-functioning enterprise, you may be obliged to advance from simple code assistants to the most advanced AI agents competent in transforming and elevating business workflows.
In this blog, we'll help you explore the journey to scale and harness agentic AI's potential like never before. Let's dive in further.
When you consider scaling your AI infrastructure requirements, you must create an environment that can evolve and grow alongside your AI project. This can be handled by handling increased volumes of datasets, more sophisticated AI models, and larger workloads without breaking a sweat. If your organizational infrastructure isn't capable enough to scale, then even the best AI solutions won't perform, limiting its output and business impact.
Let us check out this number increase as they tell the story—
According to Gartner, cloud infrastructure spending is projected to grow by 21.5% in 2025, reaching $723.4 billion. This is a significant increase from 2024, when spending was $595.7 billion
Such explosive growth is invetiable; now, leveraging cloud solutions for scalability is no longer just an option but a necessity.
Scaling up is done to reduce costs, enhance business operations, and improve decision-making capabilities. As these agents learn and evolve, they can automate routine tasks, provide real-time insights, and handle complex problem-solving with minimal human involvement.
With this scalability, organizations can now manage increased workloads without significant infrastructure modifications, ensuring they remain competitive in a constantly evolving market. Businesses can optimise processes, improve customer service, and drive growth and profitability.
When businesses deploy agents across their operations, they may often face hurdles that complicate processes. Resolving these issues is critical for the smooth and successful implementation of AI agents and maintaining the AI systems that support it over time.
Enterprises face organizational barriers in adopting AI-enabled SaaS applications that face integration complexity.
A substantial challenge is the integration of AI systems into existing platforms. Due to the extensive data requirements of AI, numerous integration tools are inadequately equipped to manage them. Consequently, businesses frequently must allocate resources to develop custom integrations or acquire supplementary solutions, which can delay and complicate the rollout process.
To guarantee that their AI systems are both practical and trustworthy, enterprises must also navigate the complexities of security, compliance and integration.
Handling data scalability is another major hurdle when scaling AI infrastructure. AI projects deal with different types of data—structured, semi-structured, and unstructured. Integrating all of these seamlessly for training and inference requires robust data governance and efficient processing pipelines, which become increasingly complex as data volumes expand.
AI projects thrive on data, but managing and scaling these datasets is no simple task hence making sure data flows efficiently can be the difference between success and failure. Data lakes can be a great stop for storing huge amounts of raw data, while data warehousing helps structure that data so it’s ready for use in specific AI applications.
The smooth operational functioning when the demands increase is must when scaling your AI infrastructure. This is where MLOps comes in(the ML variant of DevOps) bringing its principles into the machine learning infrastructure. MLOps can automate and streamline things, but scaling up MLOps comes with its own set of challenges. You need solid version control, strong collaboration between data scientists, engineers, and DevOps teams and consistent deployment pipelines. And being honest—keeping everyone on the same page as infrastructure scales which can be tough and lead to inconsistencies in model deployment.
Security is the top concern for enterprises, with 62% of practitioners and 53% of leaders highlighting issues such as data privacy, regulatory compliance, and ethical AI use. Businesses must ensure that their AI systems meet industry standards while implementing strong security measures to protect sensitive information.
Scaling AI agent systems bring in infrastructure challenges. Enterprises need to address these performance-based issues:
To overcome these challenges, executives opt in mostly for an approach that can blend in-house AI capabilities with either external or in-house solutions. This strategy allows organisations to maintain control while leveraging external expertise to address technical difficulties.
Resolving infrastructure and security issues is a must for creating a scalable and dependable AI environment. Now that you are well aware of the challenges that come when we want to scale our AI agents, we might take a look at the infrastructure required to make this thing happen.
Wondering how to optimize your current AI infrastructure for scalability? Let’s discuss your needs.
AI development is a meticulous process and for its systems to scale they require careful evaluation and considerations of various infrastructure components that ensuresreliability and optimal performance. Further we will discuss the key infrastructure requirements to an AI system architecure:
GPUs and TPUs: When you train your agentic AI and deploy them to complete specific objectives, these systems needs an infrastructure that can handle the computational demands of training and deploying AI models, thus businesses must be equipped with high-performance hardware such as Graphics Processing Units (GPUs) or Tensor Processing Units (TPUs). These resources are adept in processing large datasets while executing complex algorithms with ease.
Memory Systems: To maintain operational efficiency that can facilitate collaboration among multiple agents organizations needs to implement a cohesive memory system that integrates various data storage solutions (e.g., in-memory databases, relational databases, vector databases) these systems are functional in processing heavy data sets.
Data Pipelines: When the end goal is to manage huge amount of data, efficient data ingestion and processing pipelines are crucial for feeding AI agents with real-time data. This ensures that agents can access the necessary information to make informed decisions and thus the infrastructure must be equipped with potent data pipelines
Cloud platforms (e.g., AWS, Azure, Google Cloud) are synonymous with their utility which allows businesses to scale resources dynamically. These cloud platforms offer, good storage, on-demand computing power, and networking capabilities, enabling organizations to adjust their infrastructure based on workload requirements without significant upfront investments.
For AI agents to operate seamlessly within existing network configurations they need certain networking considerations that can enable them to function with ease. This can range from ensuring secure data transmission protocols, managing firewall permissions, and maintaining reliable connectivity to support real-time interactions.
For AI agents to be deployed there are certain protocols to be followed and adhering to organizational security policies is one of that which is critical for AI deployments. This includes implementing strict access controls, data encryption, and compliance with relevant regulations (e.g., GDPR, CCPA) to protect sensitive information.
The architectural as well as infrastructural requirements needs employing orchestration tools (e.g., Kubernetes) that can manage multiple AI workloads efficiently, allowing organizations to scale their operations without significant infrastructure changes.
AI agents needs assistance through well-defined APIs as they should be able to integrate smoothly with existing enterprise systems haslle free. This capability will ensure that agents can effectively interact with other applications and workflows within the organization.
When all the above mentioned infrastructural requirements are addressed, then businesses can effectively scale their AI agent systems, enhancing optimal performance and ensuring they remain competitive in an increasingly data-driven agentic landscape.
Adopting AI agents in enterprise and scaling them further brings opportunities and challenges. With 86% of enterprises requiring upgrades to their tech stacks, preparing for an efficient infrastructure has become a top priority.
Scaling AI agents systems, seeks significant investment. For example, a study shows that 42% of enterprises plan to develop over 100 AI agent prototypes during the scaling phase, which might need good money. These efforts will address key issues like integration, security, and infrastructure.
Tackling these challenges requires a clear focus on three main areas. Here are the three areas central to scaling AI agents:
Keeping the above factors in mind it can be assumed that the focus now must be on building strong foundations. This includes ensuring security, optimizing performance, and preparing for future scaling demands.
Enterprises looking to gain momentum as they scale and the certain need to reclaim the same with their AI agents is proof the businesses will further improve and excel.
If you are also a business looking to integrate agentic AI in your workflows or feel to level up by scaling AI agents systems scope you can check out our work. We have partnered and assisted businesses with integrating and developing AI agents that can manage and elevate operational workflows and generate revenues.
Eager to learn more about building your AI product with Codiste? Reach out to us
With careful planning and the right tools, scaling your AI infrastructure can be a manageable, even rewarding process. It’s about building an AI system that grows with your ambitions and meets the demands of a rapidly evolving industry.
Share your project details with us, including its scope, deadlines, and any business hurdles you need help with.
Countries Served Globally
Technocrat Clients
Repeat Client Rate