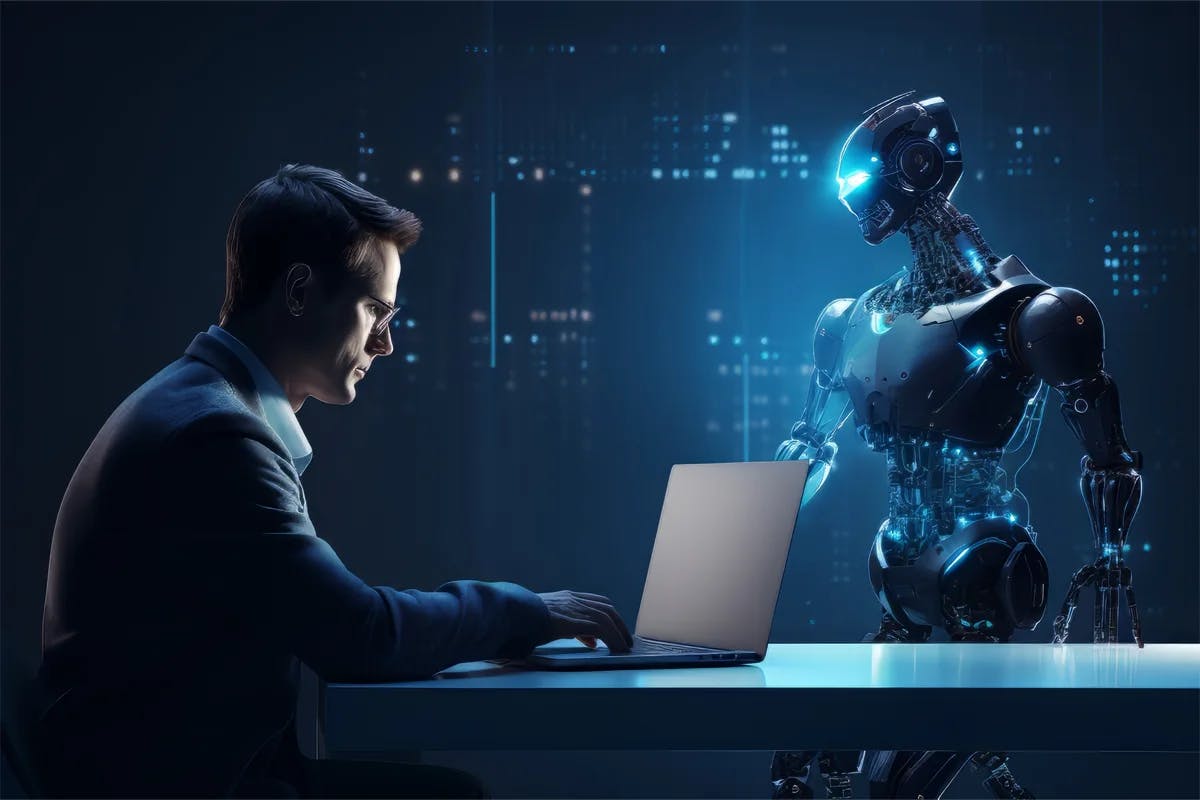
Machine learning is the most important and promising technology that is going to change many prominent industries. It provides one of the most effective tools to analyze data, generate predictions, and automate complex processes. This helps to increase productivity and innovation.
However, even though it is a promising technology, Machine Learning Development still has issues that might arise when considered by organizations to adopt it, due to the unclear strategy of implementation. This article investigates the potential obstacles that industries may face in the process of deploying machine learning tech in 2025, and it gives actual solutions and the best practices for them.
The integration of machine learning into industry workflows poses various significant challenges. The first step to overcoming these hurdles is understanding all such challenges.
One of the key elements in successful ML integration is the availability of quality data. Machine learning models depend mostly on data that is accurate and systematic. Such data ensures reliable and correct results.
On the other side, some organizations face quality-related data issues, such as the data being incomplete, missing, or biased. If a dataset is inaccurate or faulty, ML models cannot acquire knowledge as they should. This leads to unreliable and even false and misleading results.
Keeping data of a high standard can be quite a task since it usually implies investing a significant amount of time and resources in data cleaning, labeling, and organizing. Further, data quality problems might not be detected easily in the beginning. They only become evident after the ML model has been installed. Solving data quality issues at the initial stage is a prerequisite for the accomplishment of any successful ML project.
Model selection is one of the hardest things nowadays due to the great number of machine learning models available. Some models are better for specific purposes while some of them are best suited for different tasks. So when the wrong model is selected, it makes the process inefficient and the outcomes inaccurate.
Additionally, model selection alone is not enough, but a machine learning model requires intricate knowledge of the algorithm as well as the data, which makes this process slow and complicated.
This challenge will get more pronounced in 2025, as machine learning applications expand in different industries. Organizations trying to put ML into use in different areas basically must find ways of making the model process more understandable and shorter. If not, they may enter the possibility of losing time and energy on solving problems that do not lead to successful results in the end.
Machine learning development projects can be quite demanding in terms of resources, particularly when scaling them up. The computer system power should be large enough to train and operate machine learning models. This means the bigger they are, the more costs are also tied to them. In many sectors, spending on large-scale ML systems is a hurdle, especially for smaller organizations with limited funding.
In 2025, with the advancement of machine learning tools, the requirement for processing resources will grow resulting in higher expenses. Balancing performance with cost efficiency will be the key to the successful and cost-effective implementation of ML in companies. The inability to scalability ML models in a very costly manner might deter their usage in many industries.
Although machine learning technologies are becoming more and more popular, the issues of data privacy, bias, and ethical AI are likewise growing. Rules regarding data usage and privacy are constantly being updated, and machine learning models should adhere to these regulations.
Ethical issues, especially on the themes of fairness, transparency, and responsibility, are also taking the limelight in the discourse. For example, putting biased models that make discriminatory decisions into practice can be highly detrimental to users. As a result, they may become victims of unfairness or even discrimination.
Come 2025, companies are going to have to consider these ethical and regulatory issues very closely. The use of AI systems in a safe way will become a major issue. Data scientists must ensure models follow ethical and regulatory principles, besides fairness and accountability. This will be highly required by sectors like healthcare and finance.
With the increasing use of machine learning in different verticals, it is expected that the need for qualified professionals also will be on the rise. Unfortunately, the lack of machine learning professionals is prompting a situation where companies cannot have the employees needed to operate and sustain their ML systems. The discipline of machine learning requires knowledge in fields such as data science, programming, and statistical analysis—skills that may not be easily available.
The lack of skilled ML personnel might cause the projects to be delayed and the costs to be higher. The organizations might have to spend money on the training of their current staff or depend on the consultants as well.
Organizations either need to train the current AI-enabled staff or hire professional consultants in such a case. By the year 2025, companies will have to come up with ways of closing this skill gap in the workforce if they want to fully implement their ML models.
Facing challenges in implementing machine learning while executing?
The successful integration of machine learning is based not only on the use of powerful algorithms but also on diligent planning and best practices. Here are some of the best practices:
For organizations to deal with data quality issues, they should allocate funding to implement robust data management techniques. This involves procedures for cleaning, labeling, and updating data to make sure it is accurate and up-to-date. Regular data quality audits not only minimize problems but also boost the efficiency of ML networks.
By making data management a priority, organizations can establish a strong base for their own ML projects. Well-organized and credible data allows ML models to learn more effectively, which in turn leads to better outcomes.
The selection of the right machine learning model is a crucial step in the process of the implementation of desired results. It is better to move from the traditional attitude of using the most complex model to the one that better suits a specific organization's objectives and data. Simple models sometimes outperform large models in terms of being both easier to handle and interpreted with a higher degree of understanding.
To make the model selection easier, companies can create standardized guidelines that specify the criteria for the model selection. This would guarantee that the process is similar every time and the evaluation of various options is made quickly.
Creating scalable infrastructure is essential for the management of the increasing demands of machine learning. Some cloud service providers give flexible options in their applications where organizations can increase computing resources according to their needs. Also, they get to pay for only what they use. Cloud platforms also come with several tools that are specifically made for ML, thus making the management of resources and cost control easier.
Companies can develop scalable infrastructure that is easily adjustable to changing demands and also meet the expansion of their ML initiatives without causing any high costs.
Organizations should set up specific rules for the usage of machine learning considerately. This makes sure that the decision-making process involved in models is transparent enough. It should also ensure identifying and combating biases, and adhering to the regulations properly. Periodic analysis of ML models for the alignment with ethical concerns is of utmost importance in high-risk fields such as finance and healthcare.
Responsible AI practices can be used to improve processes. This is also helpful to make people feel confident and believe that the company as a whole is ethical and follows strict data privacy rules. This helps to establish a positive mutual relationship. Regulatory compliance is also ensured by constantly being updated about any changes in the regulatory environment.
In order to tackle the skills gap, companies must finance the employees they currently have to learn new skills and also hire a varied squad of ML developers. Organizing training programs or sponsoring the employees' education in learning new ML skills is a way to shun the knowledge gap. Moreover, a diverse Machine Learning development team can offer different points of view, which can result in the promotion of a creative environment and the ability to find solutions to problems.
Recruiting a machine learning development team with various backgrounds may be instrumental in preventing the emergence of biases in ML models. This can result in more inclusive and balanced solutions.
Several industries have already experienced the advantages of machine learning development by receiving useful outcomes that companies can use to make their operations more efficient:
In 2025 we expect machine learning development to disrupt sectors even more, although successful integration will come only through a wise strategy for overcoming the known problems. On one hand, there is the question of managing data quality; on the other, there is the question of ethics and the skills gap. Both technical and human aspects need to be the main focus of the organizations for successful ML integration. Through adherence to these best practices, industry players can produce the desired benefits, which are the addition of new customers and the enhancement of the operational cost-effectiveness of machine learning applications.
Businesses that opt for Codiste, a top ML development company in the USA, for their machine learning technology development will have their own customized ML solutions that perfectly match their exact needs. Through Codiste's recommendations, the companies can overcome the integration difficulties of machine learning. As a result, they can utilize this dynamic technology in a beneficial way. Contact us for expert recommendations on your next ML integration solution project.
Share your project details with us, including its scope, deadlines, and any business hurdles you need help with.
Countries Served Globally
Technocrat Clients
Repeat Client Rate