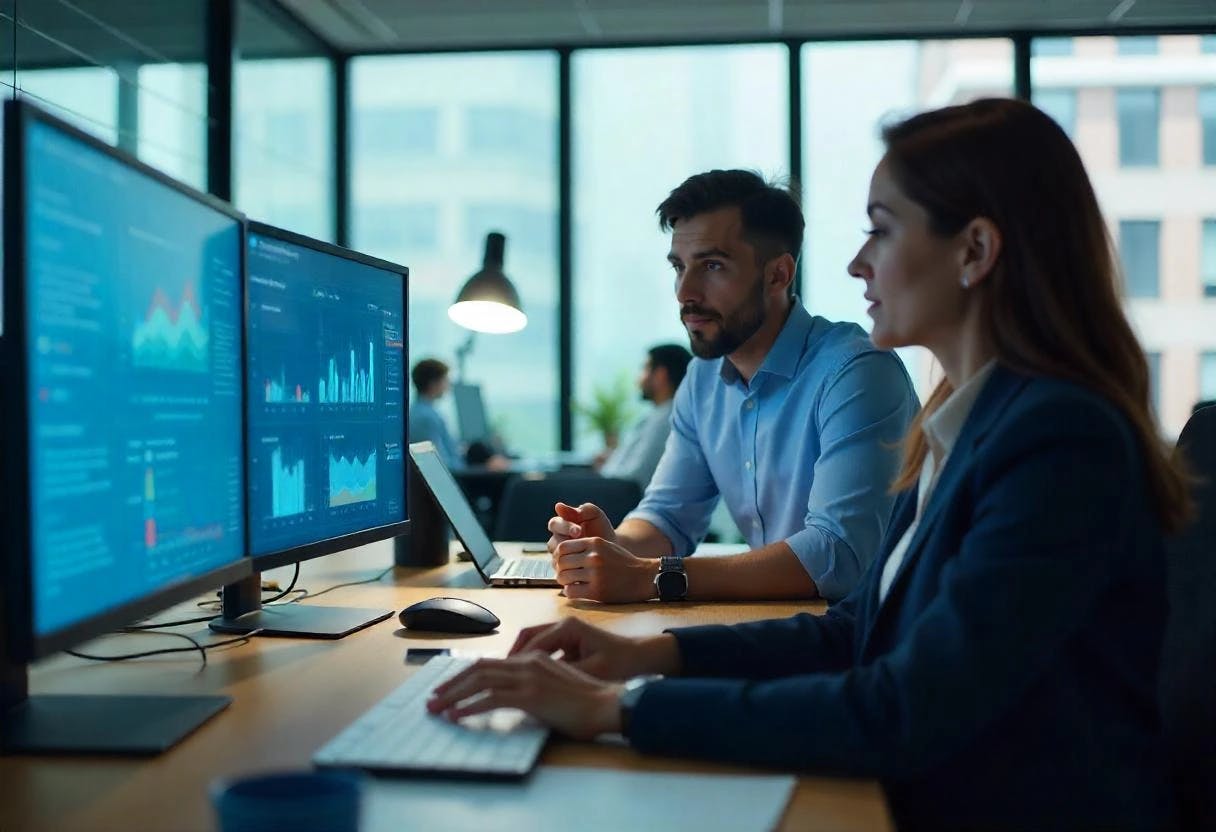
In the ever-changing field of Generative AI, pre-trained models are avant-garde tools. These pre-trained models in AI have changed how we design and use machine learning systems. They are the solid foundation that spurs creativity, reflecting vast and different datasets they've undergone detailed training on. This all happens before their use in real-life situations through a method known as fine-tuning.
Let's explain further. Pre-trained models in AI don't originate from one single task or area. They are carefully built by being exposed to a wide range of information. This first training stage gives them a core understanding of patterns and features found in the wide variety of data they deal with.
These pre-trained models in AI get better at picking up subtle differences, whether it's understanding words, recognizing images, or doing other tricky jobs.
The important thing about them is how flexible they are. They're not limited like regular models. Instead of being made for just one job, these pre-trained models in AI finish their learning stage with a wide understanding of different patterns and structures found in the data. This wide range of knowledge makes them super helpful for developers starting projects that need; creativity, adaptability, and efficiency.
In the continually changing world of Generative AI, pre-trained models serve as an essential piece, leading a major change in how artificial intelligence systems handle creativity, content generation, and solving problems. Central to this progressive role is the pre-trained model's outstanding ability to understand and hold overall patterns and knowledge from a wide array of various datasets.
Pre-trained models in AI through this immersive exposure to diverse datasets, acquire an intrinsic understanding of the intricate relationships, underlying structures, and multifaceted patterns that characterise the complexities of the real world. This depth of knowledge becomes the cornerstone of their proficiency, enabling them to transcend the constraints of singular tasks and specialise in generative creation.
Pre-trained models in AI form the base for creating different kinds of content, like making realistic pictures, writing well-connected and detail-rich stories, or dreaming up whole situations that combine believable details with creativity. Pre-trained models are an unmatched tool for this. They can understand different kinds of data, allowing them to create content that not only reflects the details of the original data but can adjust to new and unseen patterns.
Think about how picture-making pre-trained models in AI, like StyleGAN, turn pixels into attractive art. These models use what they learned about shapes, colours, and textures to create pictures. They look real but can also be better than real, showing what's new in artistic expression.
There are also pre-trained models called GPT-3 that are really good for written language. They can narrate, reply to cues, and chat, much like the broad text data they trained on. Since they are good at understanding context, figuring out feelings, and copying how people talk, they can be used in many different ways - from chatbots to summarising content.
Artificial intelligence keeps changing, with pre-trained models becoming key innovators. They provide developers with a starting point into the complex world of pre-trained models for AI development. These models, refined by extensive practice on varied datasets, power apps that exceed what we can imagine. As we explore the detailed world of generative AI model applications, it's important to highlight the leading pre-trained models that excel in creativity and solving problems.
GPT is a leader in natural language processing created by OpenAI. It is known for creating versatile language, helping in things like artificial conversation to content crafting. Its two-directional architecture lets it both understand and create contextually rich language. This makes it really strong in the areas of textual creativity. GPT is used in semantic search and can help users search for a reaction to an inquiry with just a few clicks. Rather than watchword coordinating, GPT can be used to answer complex standard language requests rapidly.
StyleGAN (Style Generative Adversarial Network) shines in the field of image creation. Known for its ultra-realistic image generation, it has gained recognition in both art and practical uses, such as facial recognition. Introduced by Nvidia researchers in December 2018, StyleGAN uses a substitute generator architecture for generative adversarial networks. In order to use the adaptive instance normalisation, it borrows from style transfer literature. The subtle control it gives over the style and features of created images has made it a popular tool for those looking to expand the limits of visual creativity. They can have amazing outcomes using the StyleGAN architecture to generate high-quality and large-resolution synthetic human faces.
BERT (Bidirectional Encoder Representations from Transformers)
BERT (Bidirectional Encoder Representations from Transformers), a creation of Google, symbolises the merging of text comprehension and setting. Its two-way method for grasping context info has bolstered its strength in jobs like resolving queries and summarising articles.
Fine-tuning pre-trained models is integral to the world of machine learning. It leads pre-trained models to adapt to specific tasks without sacrificing the precious knowledge they garnered from their initial training. Essentially, it is like a metamorphosis that perfects these models, endowing them with the extraordinary skills necessary for unique roles. This intricate fine-tuning pre-trained models process guarantees that the models become adept in designated fields while still retaining the knowledge from their extensive training.
Think of it this way, fine-tuning is like customising a pre-trained model to fit a certain job or area. Pre-trained models in Artificial intelligence are like sponges; they soak up all kinds of knowledge from many different sources. But fine-tuning pre-trained models is what makes them more than just a sponge. By fine-tuning, these models gain special skills. These skills allow the models to match up with different tasks that have certain requirements.
Picture this: a model that has learned all about language from many sources. This model knows a range of languages and all their nuances. Then, fine-tuning pre-trained models steps in. It hones this all-knowing language model so it understands a desired language even better. Not only does it understand, but it can also create meaningful sentences or come up with answers that perfectly fit the context.
Think of fine-tuning as an artist shaping a sculpture using a chisel, a type of tool. Here, the chisel is like the details in the tasks. The artist is the learning gadget, and the pre-ready model is the raw material. The sculpting or fine-tuning makes the model fit specifically for a selected task.
Fine-tuning is like a bridge. It connects what pre-trained models know generally to the specific needs of real-life applications. It makes sure these models understand the details of their tasks. It also helps them adjust to changing data types they'll face during use.
As we dive into the depths of fine-tuning pre-trained models, studying their techniques and fine points, we find a way to power up pre-trained models. This method is not only effective but also custom-made to meet the unique needs of the AI model adaptations they are programmed to help.
Think of tweaking a pre-used model as a careful, step-by-step task. You change the model's inner parts, to fit a certain task or field. This method lets the model use the basic knowledge it got from the pre-training. Plus, it molds its skills to handle the special points of a particular application.
The Essence of Fine-tuning
Think of fine-tuning as a personal touch added to an already trained model. Picture the model as an artist. This artist has lots of skills learned from many different datasets during their initial training. Fine-tuning is like an apprenticeship. Here the artist can better their skills, focusing on a specific style or technique.
Pre-training lets the model see all kinds of patterns and details from a big and mixed dataset. It learns how the data is structured and how pieces connect. But this learning isn't specific—it doesn't apply to a particular job. Fine-tuning strategies shape this knowledge. It does so by showing the model a dataset for a certain task, getting it to tweak its parameters.
Adapting to Task-specific Requirements
Think about a language model that already knows a lot about syntax, semantics, and language details in many fields. One can fine-tune this model for sentiment analysis tasks to sense feelings, through exposure to a dataset purposely built for this work. When the model faces this new information, it tweaks its internal mechanisms. This focuses on patterns and is critical in detecting feelings in text.
This adaptation makes sure that the model becomes in tune with the exact needs of the app. These pre-trained models for AI development could be used to generate clear language, make predictions, or notice patterns within a given context.
Iterative Refinement
Tweaking is usually a repeating cycle, needing an even mix of keeping the helpful facts from the previous learning phase and getting used to the details of the project data. Many rounds of working with the task data, training, and checks help the model slowly improve its grasp. Such recurring improvements make sure that the model reaches a stage where it doesn't just shine at the given job but also handles new data well.
When dealing with a model already tailored by lots of data, you need to fine-tune it for a specific task. This means you don't start from zero! You modify it slightly to perform better for a specific use. The steps? Prep your data, choose a suitable pre-existing model, tweak some parameters, and continuously watch its workings and results.
By selecting the precise model, adjusting settings, and monitoring its behaviour, experts can shape these models to be spot-on for particular tasks. This approach keeps the balance - the AI model learns new stuff, yet keeps the original learned knowledge. It's about getting good at new tasks, while still knowing the basics.
Adjusting pre-trained models is a detailed, ongoing task. It's about tweaking the model's inside settings. This helps it go from knowing a lot on a broad scale to being really good at one specific thing. In this part, we'll explain how adjusting pre-trained models works. We'll show how models can learn to switch from being general to being expert.
1.The Dynamics of Fine-tuning
2.Fine-tuning Workflow
Fine-tuning pre-trained AI models is helpful, but has its challenges. Overcoming these problems is key to top performance and successful use of the models in real-life tasks. Let's discuss some of these big issues in tweaking these models:
1.Enhanced Accuracy in Speech Emotion Recognition
Improving a broad SER dataset notably boosts the customised pre-trained AI model's skill in pinpointing emotional subtleties in speech. This effort is majorly visible in everyday instances, like customer communication and voice-operated apps. Not just heightening its capability to spot emotions, fine-tuning also magnified its adaptability to various speech rhythms and accents.
2.Efficiency Gains in Video Summarization
The process of tweaking a ready-to-use model for video summarization showed significant efficiency improvements. The pre-trained language models, at first prepared using a wide-ranging video dataset, changed their learning to concentrate on important scenes and major events from task-specific datasets. This change manifested in fewer computing resources needed to summarise videos, which makes the application suitable for immediate use situations.
In the growing world of generative AI applications use cases, tweaking pre-made models is about to experience major progress. We predict dramatic improvements in adaptability, efficiency, and morals in the future of fine-tuning. Take a peek at the expected trends that will mould the future of fine-tuning for Generative AI:
Fine-tuning pre-set models is a game-changing method for improving creative AI uses. Developers can make use of the might of pre-set models and modify them to suit their unique application needs. The hurdles in fine-tuning are eclipsed by the perks, as demonstrated by generative AI app use case examples and continuing progress in this field.
At Codiste, we know how essential the adjustment process is to fully activate pre-trained models for Generative AI uses. Our advanced tools assist coders in dealing with the trickiness of this process, promising top functionality and effectiveness in their work. Book a call with us to be part of an exciting venture where imagination pairs with tech, and together, we can mould the future of generative AI.
Share your project details with us, including its scope, deadlines, and any business hurdles you need help with.
Countries Served Globally
Technocrat Clients
Repeat Client Rate