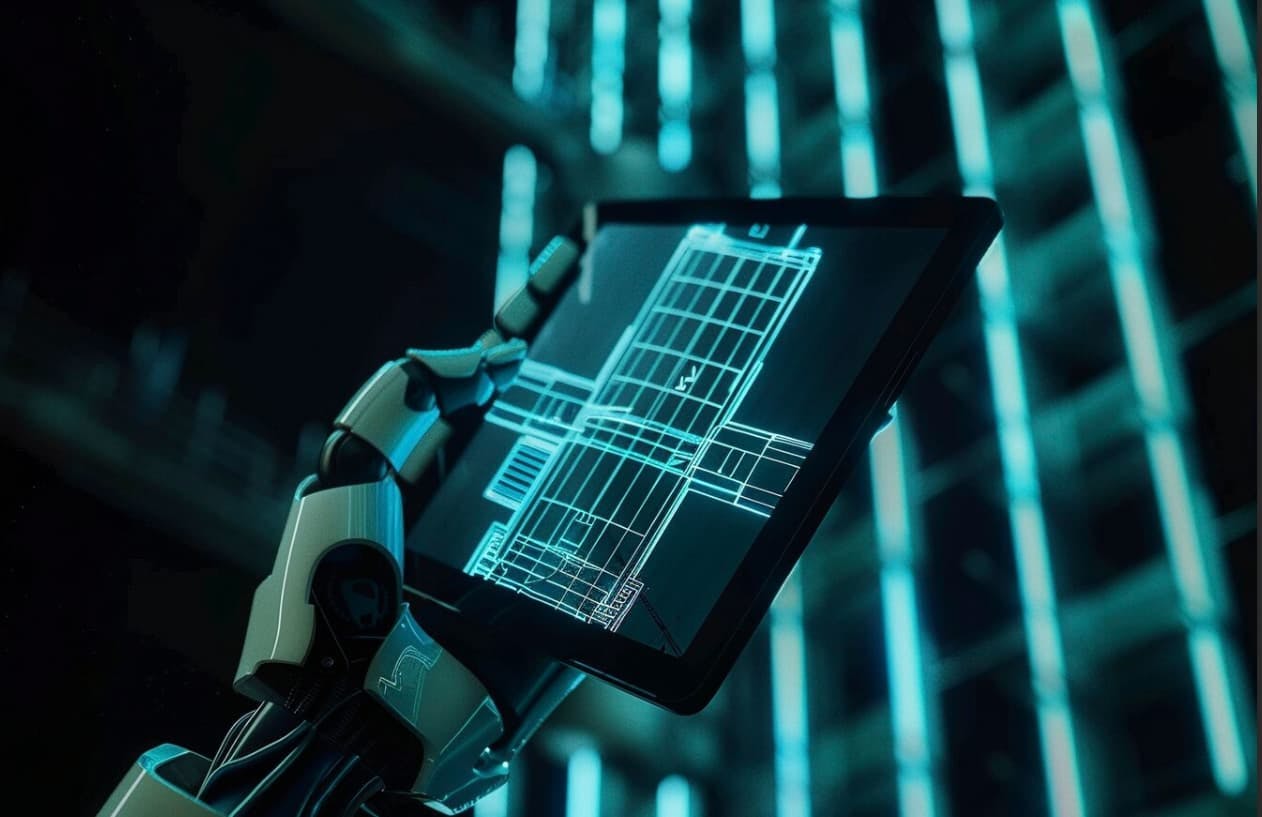
,
,
This might be an understatement to say that sooner autonomous systems will independently execute tasks and resolve issues in the early 20s. But with the advent of machine learning capabilities and natural language processing, humans could comprehend and engage with their surroundings and build intelligent agents that can act part-human, part-machine. With this exclusive guide, we will examine the nature of AI agents, how these AI Agents for enterprise automation function, and their revolutionary effects across various sectors.
We have talked a lot about how AI agents are crafted to function independently or provide support to users in completing tasks with greater efficiency in our previous blogs as well. Drawing upon the capabilities of generative AI, absorbing context and learning from interactions with users allowing them to become highly adaptive and flexible within enterprise automation. These systems possess intelligence that enables them to perceive their surroundings, decide on a course of action, and execute measures necessary for achieving predetermined objectives.
AI in enterprise automation harnesses sophisticated technologies like machine learning, natural language processing (NLP), computer vision, and artificial intelligence (AI); these agents exhibit intelligent behavior. This equips them with the ability not only to carry out routine actions but also to address intricate challenges requiring problem-solving skills.
Intelligent business automation is revolutionizing various sectors by streamlining workflows related to automation processes. This leads to cost reductions in operations while simultaneously boosting overall productivity.
There exists a diversity of AI agents, each distinguished by their specific attributes and capabilities.
These are the most basic types of AI agents. They operate purely based on current perceptions, ignoring the rest of the environment's history. Reflex agents follow predefined rules and react instantly to specific conditions.
These agents are an upgrade from simple reflex agents. They maintain an internal state that depends on the perceived history, helping them make better decisions based on both the current input and previous experiences.
These agents make decisions based on achieving specific goals. They evaluate different possible actions by predicting their outcomes and choosing the action that will bring them closer to their goal.
Utility-based agents go a step beyond goal-based agents by not only aiming for a goal but also optimizing for the best outcome. They assess different options by assigning them utility values and choosing the most valuable one.
Learning agents are adaptive systems that can improve their performance over time. They learn from experiences, adjust their behavior, and evolve without explicit programming for every situation.
Autonomous agents can operate independently in dynamic environments, making complex decisions without human intervention. Multi-agent systems involve multiple autonomous agents that collaborate (or compete) to solve complex problems.
Selecting an appropriate AI agent tailored to particular business requirements guarantees proficient execution of the tasks at hand.
The design of these agents is structured with the intent of AI in enterprise automation architectures serving as a master plan for how these advanced systems function independently and adjust to their surroundings. It includes various fundamental elements that allow AI agents to sense data, analyze information, and carry out decisions with efficiency. Recognizing these elements is crucial in understanding the intricate processes that facilitate the operation of AI agents.
Equipped with Large Language Models (LLMs), among other tools, agentic AI possess the ability to autonomously explore environments, devise strategies, engage in self-reflection, and exhibit behaviors aimed at fulfilling specific objectives within complex settings.
Three critical components—Large Language Models (LLMs), integration of various tools, and memory mechanisms—work together, forming an integrated framework empowering AI agent to tackle tasks on their while adjusting seamlessly when faced with new obstacles.
The perception module collects environmental data and makes sense of it, offering the agent situational awareness that helps it comprehend and respond to what’s around it.
By utilizing a modular design approach along with incorporating machine learning advancements, AI agents are equipped to adjust more effectively when faced with new challenges.
The decision-making component analyzes various alternatives and selects the optimal strategy, employing sophisticated algorithms and structures to guarantee that the AI agent successfully achieves its objectives.
By incorporating machine learning, this decision-making element can perpetually refine its tactics using newly acquired information and experiences.
The decision-making module informs the action component, which then performs tasks and interacts with its surroundings to enact chosen actions.
In contexts such as autonomous vehicles, this action component is responsible for managing pivotal operations, including maneuvering the steering wheel, operating brake systems, and regulating acceleration—underscoring its importance in the structure of an AI agent.
Is business automation your goal? We can help you achieve that.
The new architecture for enterprise automation is modified with constant understanding and upgrading of current systems. These autonomous agents in business are made and characterized by a multi-layered framework that integrates AI agents with existing systems to significantly enhance operational efficiency and decision-making processes.
To summarize, this architecture not only aligns with an organization’s goal to streamline AI agents for enterprise automation but also empowers organizations to leverage AI capabilities for enhanced decision-making and operational efficiency.
AI agents are critical in bolstering the operational efficacy of companies by employing a variety of technologies to refine operations. They harness specially crafted algorithms and structures that have been developed to enhance digital workflows, establishing themselves as essential elements within contemporary corporate settings. The incorporation of AI agents into business infrastructures facilitates marked advancements in productivity, dependability, and comprehensive functioning.
These entities possess the capacity for learning and adaptation through their interaction with unstructured data—this attribute augments their ability to automate effectively while maintaining their utility within ever-changing circumstances. Achieving success hinges on adopting straightforward, modular patterns over intricate systems and underscores the need for thorough testing methods to ensure consistent performance in vital applications.
Demonstrating flexibility and competency, AI agents are actively employed across various sectors, such as finance, healthcare, and manufacturing. They excel at automating mundane activities while simultaneously elevating customer experiences.
Incorporating AI agents into business processes results in considerable enhancements to operations and decreases in costs. Through the seamless integration of various enterprise systems, these AI agents promote efficient workflows and enable complete automation from start to finish. Robotic Process Automation (RPA) replicates human behaviors, executing repetitive tasks with enhanced efficiency, accuracy, and robustness.
AI-powered agents can streamline intricate workflows that once necessitated direct human involvement. This elevation in productivity shifts the focus of human workers towards more strategic roles and activities that add value. By interfacing smoothly with pre-existing systems, these AI tools improve existing business processes without causing any interruptions.
By efficiently managing inquiries and scheduling, AI agents markedly enhance the experience for customers by curtailing wait times for responses. In industries such as healthcare, these agents are instrumental in offering bespoke recommendations for treatment, which can substantially improve patient health results.
By efficiently managing inquiries and scheduling, AI agents markedly enhance the experience for customers by curtailing wait times for responses. In industries such as healthcare, these agents are instrumental in offering bespoke recommendations for treatment, which can substantially improve patient health results.
AI agents contribute vitally to elevating customer satisfaction and fostering loyalty through their provision of responsive and dynamic services.
Agents equipped with sophisticated AI technologies, including large language models, are capable of executing informed decisions and handling complex tasks autonomously with limited human intervention. The application of computer vision technology empowers these AI agents to interpret and comprehend visual data, which is crucial for functions such as identifying objects and analyzing scenes.
By combining these cutting-edge technologies, the proficiency of AI agents in dealing with intricate tasks effectively is significantly improved across a wide range of uses.
Building effective AI agents for enterprises involves several key considerations, including security, scalability, and integration. Implementing strong security measures is essential to protect sensitive data and comply with regulations like GDPR. Modular design and reusable components simplify AI agent development, making it easier to scale and adapt to different enterprise needs.
Scalability issues may arise when AI agents manage high request volumes, necessitating cloud resources and distributed computing. Addressing employee resistance to AI adoption through training and communication can mitigate fears of job loss and foster collaboration.
Integrating AI solutions into current systems in a smooth manner guarantees that they will augment business operations. Using of modular architectures and the ability to interoperate means that AI technologies can be easily incorporated into established work patterns.
Such incorporation results in heightened operational effectiveness, diminished obstacles within procedures, and more optimal use of resources.
It is crucial to employ robust security protocols to preserve the reliability and credibility of AI systems. This includes adopting secure coding techniques, utilizing encryption, and employing anomaly detection to safeguard confidential information.
Developing AI systems with explainability fosters confidence and cooperation among users and the AI by guaranteeing clear visibility into the workings behind AI-driven decisions and behaviors.
Researchers are investigating sophisticated learning strategies like few-shot learning and reinforcement learning to develop more intelligent AI agents. Few-shot learning is designed to enhance the capability of AI to learn from a small amount of data, thereby quickening and streamlining the overall process of acquiring new skills. These methods diminish the need for extensive datasets, accelerate the rate at which AIs learn, and facilitate their ability to manage increasingly complex tasks with less difficulty.
Integrating AI agents into systems is not without difficulties, as it involves dealing with issues such as bias, a lack of transparency, and the need for ethical accountability. Crafting dependable and flexible AI systems presents a significant challenge. Overcoming these obstacles is imperative to ensure their successful utilization in various industries. Addressing these concerns typically requires the establishment of equitable practices alongside enhancements in clarity regarding how AI operates and boosting the agility of AI technologies.
When organizations concentrate on developing automation solutions that tackle these challenges head-on, they are better positioned to surmount barriers linked to deploying AI agents. To cope with evolving data trends while preserving dependability necessitates ongoing vigilance through persistent supervision and updating training for AIs.
Testing approaches that are both robust yet modularly constructed — favouring simplicity over complexity — play an essential role when confirming the trustworthiness of artificial intelligence agents within vital operations.
To guarantee the ethical implementation of AI agents, they must conform to societal norms and legal requirements while confronting challenges like bias, fairness, transparency, and accountability. Conducting routine assessments and accumulating a varied range of data are vital steps in mitigating biases and upholding fairness within these AI systems.
Effective project management, clear documentation, and robust testing strategies are essential for handling complexity in the development of AI agents. Employing modular design principles and reusable components also aids in managing complexity by simplifying updates and maintenance of AI systems.
For development teams navigating through the intricate nature of AI agent systems, establishing comprehensive documentation is crucial.
AI systems need to be reliable, especially when they are involved in making significant decisions and taking vital actions. These AI agents can execute complex tasks quickly and precisely, which frees up personnel to concentrate on more sophisticated work. To ensure that these systems' reliability remains intact, engaging in ongoing enhancement and implementing strong testing methodologies is essential.
AI agents in enterprise automation have revolutionized how businesses can significantly automate and enhance their efficiency in operations, elevating customer interactions and adeptly handling intricate tasks. These intelligent assistants utilize architectures for AI workflow automation, focusing on executing various functions with intelligence. These autonomous AI agents underpin essential elements like perception systems for understanding inputs, decision-making mechanisms for analytical processes, and action modules that enable them to carry out their tasks effectively.
Successfully incorporating AI agents within enterprise frameworks requires meticulous planning around integration strategies, robust security measures and progressive learning methods. It’s imperative to address ethical issues conscientiously while managing complexity and ensuring reliability to integrate AI agents seamlessly into business settings.
Organizations aspiring to excel must invest in ongoing enhancement initiatives alongside modern-day technological advancements. In that respect, we at Codiste have substantially curated capable AI agent development solutions that can fully leverage the capabilities offered by AI agents in meeting corporate objectives efficiently. To know more, contact us now.
Share your project details with us, including its scope, deadlines, and any business hurdles you need help with.
Countries Served Globally
Technocrat Clients
Repeat Client Rate