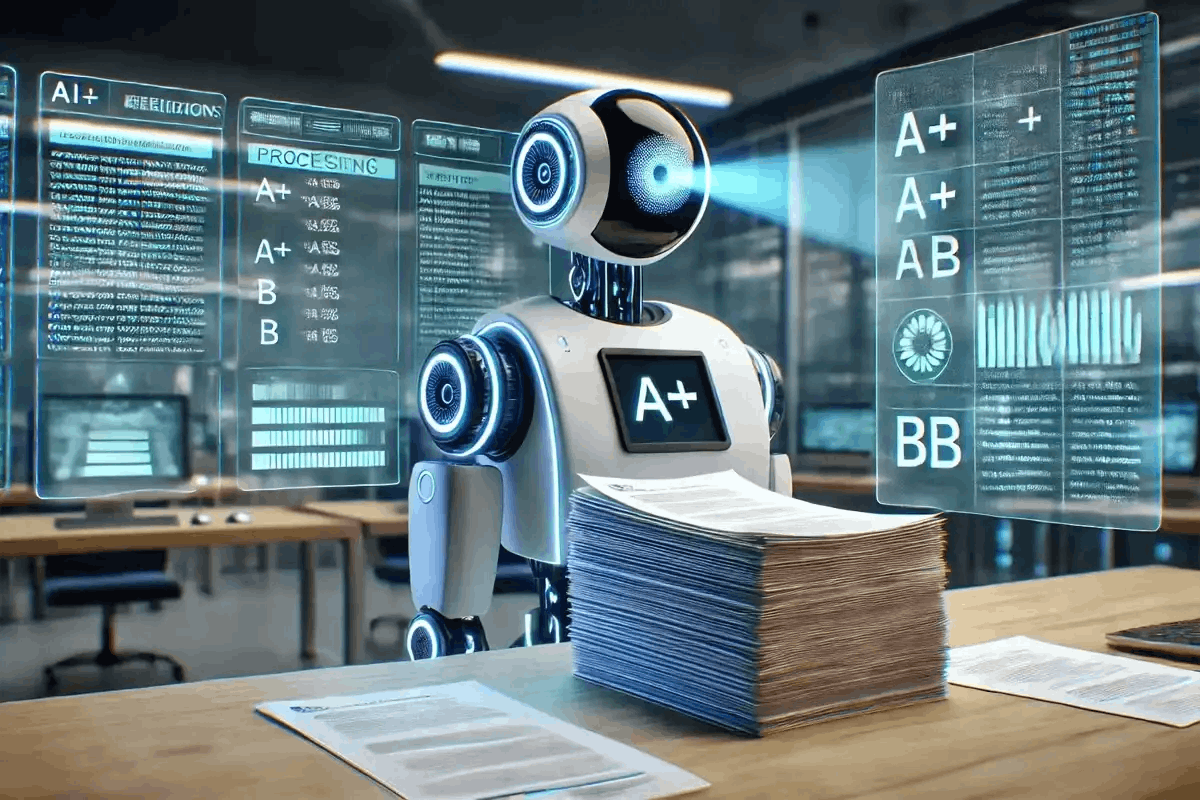
AI is increasingly becoming popular due to its capacity to automate mundane tasks, make informed judgments and speedy decisions. The AI market is expected to reach US$826.70bn by 2030. Despite the promising aspects, these AI algorithms sometimes lack the characteristics of human-like qualities.
It is commonly wondered whether there is a way to develop machine intelligence that will be more autonomous, self-governing, intelligent and able to meet the complex nature of various types of problems. A new field that has been evolving as a solution is known as Agentic AI. This advanced AI development technology promises to deliver more effective, smarter and self-dependent machines that can make judgments or decisions that best suit each situation.
But what do companies do when they do not have the benefits of Agentic AI? In this article, we will look into the difficulties that organizations face if they don't use agentic AI. We will also explore reasons why companies need to use Agentic AI to remain competitive in an era of continuous technological changes.
Usual AI models are often constrained by certain, predefined tasks and depend largely on supervised learning and human intervention. Mainly, these systems are great at performing repetitive and data-heavy tasks. However, they lack the intelligence to operate independently in dynamic situations. They can identify patterns, automate processes and generate predictions based on historical data, although they do it reactively rather than proactively.
The limitations of traditional AI systems come in several ways over reliable Agentic AI:
In the absence of the ability to work independently and make quick decisions, businesses end up with inefficiencies, higher operational costs and limited scalability.
Rapidly changing business landscapes require making the right decision on time and with accuracy. Most of the traditional AI models are mainly based on extensive data training and long processing time which could bring out insights that delay strategic decisions. Moreover, these systems typically rely on human interaction for either validation or calibration of the results which is why it takes more time.
Example:
Imagine a bank that deals with real-time trades. It is likely that the bank will be unable to adapt to the market's changing conditions and face the situation of missed opportunities and financial losses, in case it doesn't use agentic AI.
Companies often use traditional AI models which lead to high operational costs because of the continuous need for human interaction. The act not only leads to the lengthening of the processes but also to the increase in employment. This kind of manual operation of the systems (managing and maintaining the algorithms and the associated data inconsistencies) makes it even more costly to handle.
Example:
In the manufacturing industry, predictive maintenance systems based on machine learning could be useful only if the operators update the programs according to the equipment's wear and tear. Much of the time is wasted in maintenance operations which leads to higher costs of operations.
Traditional AI systems are designed for specific and narrowly defined use cases. The unavoidable limitation in this approach causes issues in scaling these solutions from one use case to the other. Organizations may find it extremely difficult to have AI-enabled operations in more than one department due to the excessively high number of customized applications invested. Instead, if the same AI could be adapted to all areas, businesses would be able to have universal operations.
Example:
AI customer support is a great tool for an e-commerce platform, but it might not be scalable to other areas like inventory management or personalized marketing, if it is based on a traditional AI approach. Each new application demands a particular system thus, complicating the network and reducing efficiency.
One of the most important difficulties that new AI is dealing with is its lack of efficiency in handling unstructured data such as text, images and videos. These are actually more than 80% of all the data produced today. Conventional AI systems frequently need massive preprocessing to make unstructured data compatible, consequently limiting their capacity to get insights from various data sources.
Example:
Doctors, hospitals, or health systems that would like to dig into and extract data such as patient records, scans, or handwritten notes for diagnostic purposes in the absence of machine learning tools (Agentic AI) can run into delays and missed informants.
Now, customers need more of a personalized approach. However, many of today's AI cannot deal with such a thing to produce real-time, context-aware interactions. In general, those systems contribute from the historical data to make suggestions and they are, in turn, outdated or not fixed to the current situation.
Example:
A streaming service deploying regular AI is likely to suggest content based only on the user's prior viewing records and hence, potentially ignoring their live behavior patterns. This then causes the users to be less interested and then lowest customer satisfaction.
Agentic AI, a new frontier for AI technology, sets a goal to deal with the problems of traditional AI systems. Unlike Conventional AI, Agentic AI utilizes self-directed agents that can sense their surroundings, make decisions and act with little or no human intervention. Such an autonomous nature contributes to creativity in dealing problems and flexibility in real-time scenarios.
The Agentic Workflows are a sophisticated system and iterative process. They are designed to improve the effectiveness of enterprise processes. Agentic Workflows use AI agents to integrate with the business settings. Agentic AI workflows collaborate and execute complex tasks with high accuracy.
The AI Agentic development must go through several important steps to guarantee that they can function on their own. Here is a simplified process explained:
Firstly, you should choose the environment that you will put the agent in to create an AI agent. You can decide if you will integrate the AI agent into an app, a website or any other system.
AI agents require good-quality data to be trained. This step consists of the required data collection from different sources, data cleaning and data organizing. The data is then made available in a format that AI can interpret that will ensure correct AI operation.
Then the team comes up with a design of the system that the AI agent works on. One of the important issues involved here is the right technologies and models to use.
Then the AI agent is trained with the data that has been prepared. It uses this information in the process of discovering patterns and making AI-based decisions. The developers guide the model to increase its accuracy and thus ensure high performance in real-world settings.
The AI agent is evaluated first in a closed environment before it is deployed into production. The simulated environment aids in the identification of issues and the agent's capacity to perform various operations such as customer queries or basic process automation smoothly.
Finally, AI is added to the real world. One of the key ways to ensure that a newly introduced AI agent is successful is to never take monitoring for granted. It is important not only to check his performance but also to see whether or not he has learned from new data. This is exactly how AI agents stay relevant and operate smoothly.
Agentic AI makes operations more efficient by automating complex decision-making processes that ultimately, increases productivity. It decreases human involvement and thus, it helps to lower costs. Businesses can efficiently allocate resources to achieve the organization's vision in the best possible way driven by innovation.
Example:
In supply chain management, Agentic AI autonomously finds the best routes, adapts to real-time situations and controls the inventory with no human intervention. As a result, the deliveries are faster, the fuel cost is less and the stockouts are reduced.
Agentic AI can boost the quality of the customers' experiences through highly individualized interactions drawn from real-time data. Each customer's behavior at the moment is analyzed by the system, according to which, interactions are highly relevant and engagement and satisfaction are improved.
Example:
Retailer firms can utilize Agentic AI to formulate flexible pricing techniques, customized marketing campaigns and adaptable product recommendations. This would lead to higher conversion rates and customer loyalty.
One of the key strengths of Agentic AI is its ability to forecast and reduce risks even before they affect the company. Through the continuous tracking of different data sources, these systems can detect probable problems and operate independently in carrying out corrective measures.
Example:
In the financial services sector, company-specific AI ‘agents’ can recognize unusual behavior in transactions and report possible fraud. This in turn minimizes financial risks and ensures compliance with regulatory standards.
Contrary to the previously used AI method, which most often needs tailored solutions for the mentioned application, Agentic AI provides scalability for multiple fields. The capacity to adjust itself and the acquired knowledge autonomously makes it applicable to numerous sectors, including healthcare, finance, manufacturing, retail and more.
Example:
A company can adopt Agentic AI technology to streamline the whole process from customer service chatbots to robotic process automation. And thus, having a holistic and scalable AI strategy.
As we move deeper into the digital era, the drawbacks of traditional AI technologies come into the picture. Companies that are still sticking with passive, rule-based AI systems expose themselves to risks of losing their positions in the constantly developing market. The inefficiencies of decision-making processes, high operational costs, limited scalability and poor customer services have become too prohibitive to neglect.
Agentic AI would be a smart solution as it deals with the problems through its own self-reflective features and contextual understanding. Integrating this technology in their operations businesses can reach new heights of efficiency, innovation and competitive advantage. AI for business automation and the creation of intelligent, adaptive systems can function autonomously.
Businesses while trying to eliminate the limitations of traditional AI started adopting Agentic AI which has great potential, Codiste is the most trusted AI development Company in the USA for developing agentic AI based on specific use cases.
Codiste with 10+ years of experience and a skilled team of AI professionals, having expertise in the development of innovative AI systems that fulfill the needs of different industries. Codiste's expertise lies in the most advanced technologies such as machine learning (ML), natural language processing and decision-making algorithms.This helps us to create systems smarter and flexible enough to cope with minimal human intervention.
Codiste is helping organizations to provide better customer experiences, increase operational efficiency and grow in a scalable way with great knowledge and skills in building advanced autonomous AI solutions. Companies adopting Codiste's innovative Agentic AI development services can get more adaptive and intelligent systems.
Share your project details with us, including its scope, deadlines, and any business hurdles you need help with.
Countries Served Globally
Technocrat Clients
Repeat Client Rate